Machine Learning: A Solution to Today's Security Threats & One Step Closer to AI
Algorithms can identify threats that have been missed by traditional security mechanisms.11/19/2014 11:44:00 AM
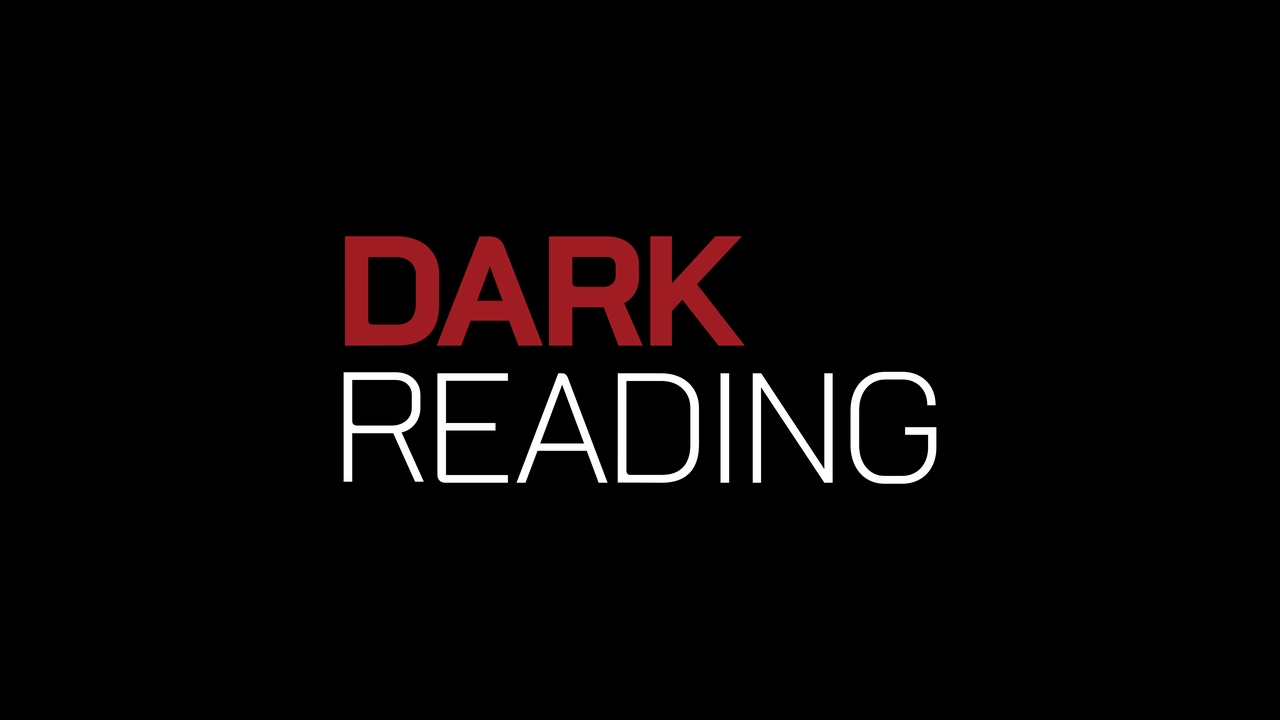
Artificial intelligence is becoming a popular term loosely used whenever a new system is able to come up with high probabilistic results for solving a specific problem. Although it may be very accurate when providing answers to a specific problem, we’re still far from creating a truly self-conscious entity.
Machine-learning algorithms can solve problems that we currently cannot address using conventional methods. Chances are that 99.97% of the time these algorithms can identify threats that were missed by traditional security mechanisms. Because they exhibit some form of engineered statistical intelligence, it’s safe to assume that such algorithms, in conjunction with other technologies, could be used to explore advances in artificial intelligence.
Protecting the abundance of Internet-connected devices has become a daunting task -- one that can be overcome by using self-learning algorithms and technologies that can hammer even previously unknown threats.
For instance, imagine feeding such algorithms with information about known malware samples and security vulnerabilities to identify yet-unknown threats. By observing patterns and facts, security-centric machine-learning algorithms can derive statistical inferences leading to positive identification of new and unknown threats. While this is not Hollywood-style artificial intelligence, these systems succeed where traditional approaches fail. It’s important to realize that you cannot use a single all-knowing machine-learning algorithm when talking about security. Having multiple systems that constantly crunch specific types of data on various timespans is key to augmenting security and neutralizing exotic threats.
The silver bullet that can solve any type of problem is actually more like a silver shotgun shell -- a sum of systems. For this reason, when sticking to a particular field of interest, say, detecting advanced threats, engineering automated self-learning algorithms that can draw probabilistic outcomes based on analyzed data sets is highly efficient and accurate.
Using the brain paradigm to describe these automated systems makes sense when talking about the learning capabilities of such algorithms. Although the analogy is somewhat misleading from an academic point of view, it might bring perspective. If the human brain can deal with cluttered information, such as object recognition in images and their relation with each other, machine-learning algorithms can also be trained to individually identify objects, but without the advanced inference capabilities of human brainpower. Although they cannot currently answer questions, such as “How do I feel when looking at those balloons?” they are great at extrapolating statistical probabilities based on previous knowledge and answering questions such as “How many balloons are there?” or “How many people are holding balloons?”
Consequently, there is a constant need to develop and tweak these algorithms, especially since current statistics show that more than 12 billion devices will be connected to the Internet by the end of 2014, according to Strategy Analytics. Imagine a world where any device may become a target, where your microwave will suddenly start sending spam or your refrigerator will place bogus food orders. Now imagine having systems that understand how threats behave when attacking any type of device or operating system.
Although current security-centric machine-learning algorithms are far from taking over the world, Skynet-style, they are more than capable of thumping advanced security threats and protecting Internet-of-Things devices.
About the Author
You May Also Like