How Attackers Use Machine Learning to Predict BEC Success
Researchers show how scammers defeat other machines, increase their success rate, and get more money from their targets.
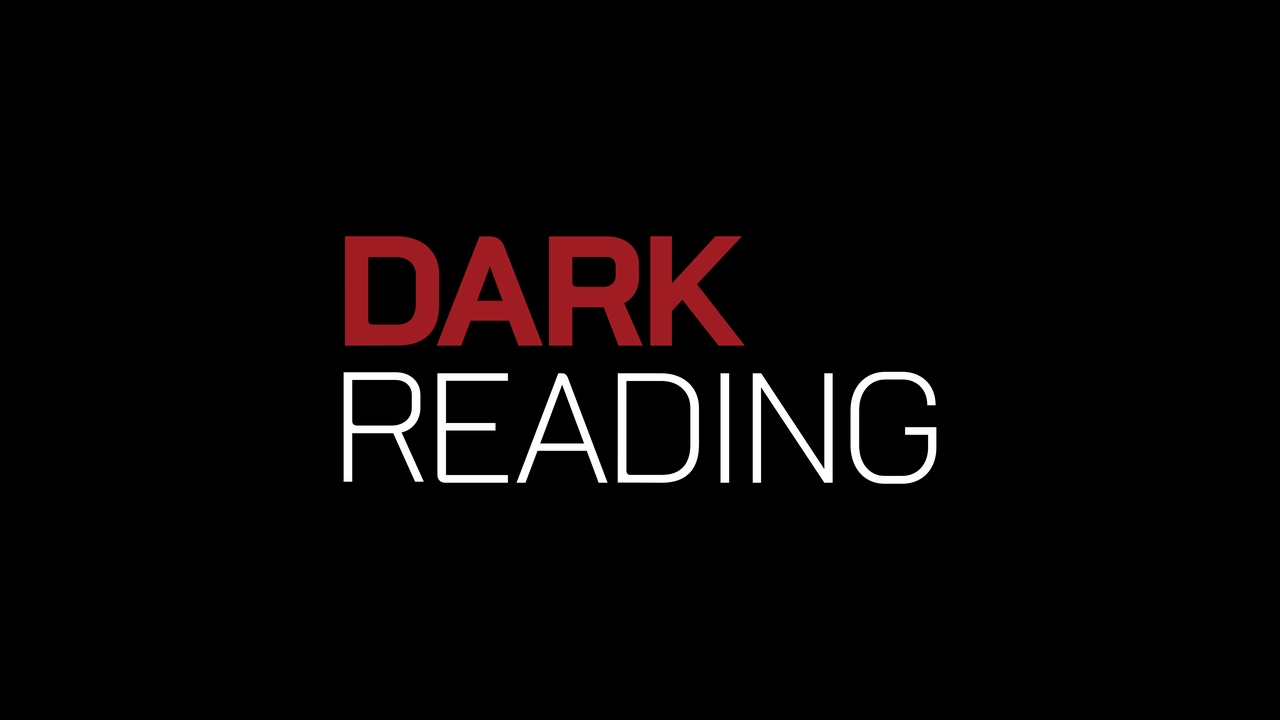
BLACK HAT USA - Las Vegas - Researchers from Symantec demonstrated how threat actors can employ machine learning models to drive the success rate of business email compromise (BEC) attacks.
BEC scams are targeted attacks on high-level executives. Attackers rely on social engineering to craft emails and convince execs to perform financial transactions, such as wire transfers, on short notice. The more a victim trusts a fraudulent email, the more likely an attack will succeed.
These scams have targeted more than 400 organizations and caused more than $3 billion in losses, said security response lead Vijay Thaware during the presentation. Attackers exploit three "defects" in human psychology: fear, curiosity, and insecurity.
BEC doesn't require a lot of funding, and most of the information attackers need is available for free online. Twitter, LinkedIn, and Facebook give a well-rounded picture of targets' lives. Company websites reveal corporate hierarchies, names of C-suite execs, and the amount of time each has been with the organization, all information that could be useful to attackers.
"It's all about how you present yourself over the Internet," said Thaware. "This data can reveal many things about us."
To illustrate his point, he presented a screenshot of a basic Google search: "chief financial officer" + "email." It was an easy and effective way to get execs' contact information, and in some cases their email addresses were available directly from the results page.
Ankit Singh, threat analyst engineer, explained how this reconnaissance and profiling prepares threat actors to launch BEC attacks. They can use machine learning to increase the success rate of access and get more money from their targets.
"Machine learning can help the attacker to bypass signature-based detection systems," he explained. "It can be used to predict various outcomes of new data based on patterns of old data." These models can also defeat other machines and anti-spam telemetry, he added.
Singh said this project involved supervised machine learning. In his demonstration, he showed how emails sent to BEC targets were marked as a "success" if the attack worked and "failure" if it didn't. The demo included targets' personal information like age, sex, number of LinkedIn connections, and number of followers and posts on Twitter.
All of this personal information was fueled into the training model, which could make predictions about whether an attack would be successful. If the attack worked, its information would be fed back into the model and improve the accuracy for future attacks.
"We feed data back into the model so the machine can learn what kind of profile is not attackable," said Singh.
He emphasized the importance of timing during a BEC attack; threat actors can use targets' schedules to plan their attacks on organizations. When they know who is doing something at a specific time, they can better plan when he would send an email and what he might say.
Singh demonstrated this idea, for example, an executive traveling to an event, and showed how the Twitter timeline, keynote plan, and travel plan could be used to indicate when he might be in transit or working.
To make their fraudulent email more believable, attackers can register domain names similar to those of the companies they are trying to imitate. This can be done for little money and effectively trick individuals and organizations, he explained.
Singh advised his Black Hat audience to be "very, very suspicious" when replying to emails. More than enough of their personal data is available publically and can be used for social engineering. As attackers start to label successful and unsuccessful attacks, their model can better determine when their actions will work.
Read more about:
Black Hat NewsAbout the Author
You May Also Like