4 Ways to Handle AI Decision-Making in Cybersecurity4 Ways to Handle AI Decision-Making in Cybersecurity
As evolving cyber threats force security teams to adopt AI to automate workflows, we ask how the relationship between humans and AI will pan out.
February 9, 2023
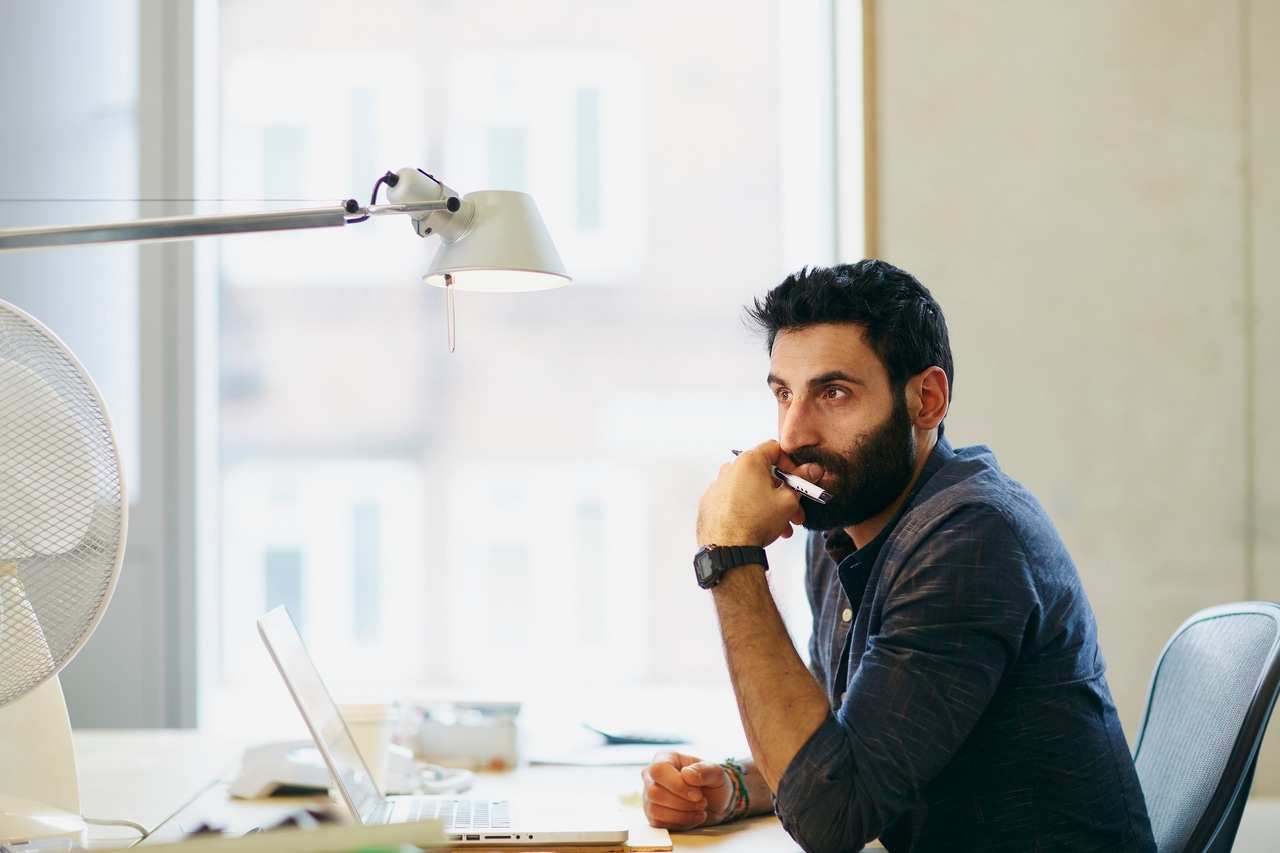
The scale of cyberattacks that organizations face today means autonomous systems are becoming a critical component of cybersecurity. This forces us to question the ideal relationship between human security teams and artificial intelligence (AI): What level of trust should be granted to an AI program, and at what point do security teams intervene in its decision-making?
With autonomous systems in cybersecurity, human operators are raising the bar of their decision-making. Instead of making an increasingly unmanageable number of "microdecisions" themselves, they now establish the constraints and guiderails that AI machines should adhere to when making millions of granular microdecisions at scale. As a result, humans no longer manage at a micro level but at a macro level: Their day-to-day tasks become higher-level and more strategic, and they are brought in only for the most essential requests for input or action.
But what will the relationship between humans and AI look like? Below, we dissect four scenarios outlined by the Harvard Business Review that set forth possibilities for varied interaction between humans and machines, and explore what this will look like in the cyber realm.
Human in the Loop (HitL)
In this scenario, the human is, in effect, doing the decision-making and the machine is providing only recommendations of actions, as well as the context and supporting evidence behind those decisions to reduce time-to-meaning and time-to-action for that human operator.
Under this configuration, the human security team has complete autonomy over how the machine does and does not act.
For this approach to be effective in the long-term, sufficient human resources are required. Often this would far exceed what is realistic for an organization. Yet for organizations coming to grips with the technology, this stage represents an important steppingstone in building trust in the AI autonomous response engine.
Human in the Loop for Exceptions (HitLfE)
Most decisions are made autonomously in this model, and the human only handles exceptions, where the AI requests some judgment or input from the human before it can make the decision.
Humans control the logic to determine which exceptions are flagged for review, and with increasingly diverse and bespoke digital systems, different levels of autonomy can be set for different needs and use cases.
This means that the majority of events will be actioned autonomously and immediately by the AI-powered autonomous response but the organization stays "in the loop" for special cases, with flexibility over when and where those special cases arise. They can intervene, as necessary, but will want to remain cautious in overriding or declining the AI's recommended action without careful review.
Human on the Loop (HotL)
In this case, the machine takes all actions, and the human operator can review the outcomes of those actions to understand the context around these actions. In the case of an emerging security incident, this arrangement allows AI to contain an attack, while indicating to a human operator that a device or account needs support, and this is where they are brought in to remediate the incident. Additional forensic work may be required, and if the compromise was in multiple places, the AI may escalate or broaden its response.
For many, this represents the optimal security arrangement. Given the complexity of data and scale of decisions that need to be made, it is simply not practical to have the human in the loop (HitL) for every event and every potential vulnerability.
With this arrangement, humans retain full control over when, where, and to what level the system acts, but when events do occur, these millions of microdecisions are left to the machine.
Human out of the Loop (HootL)
In this model, the machine makes every decision, and the process of improvement is also an automated closed loop. This results in a self-healing, self-improving feedback loop where each component of the AI feeds into and improves the next, elevating the optimal security state.
This represents the ultimate hands-off approach to security. It is unlikely human security operators will ever want autonomous systems to be a "black box" – operating entirely independently, without the ability for security teams to even have an overview of the actions it's taking, or why. Even if a human is confident that they will never have to intervene with the system, they will still always want oversight. Consequently, as autonomous systems improve over time, an emphasis on transparency will be important. This has led to a recent drive in explainable artificial intelligence (XAI) that uses natural language processing to explain to a human operator, in basic everyday language, why the machine has taken the action it has.
These four models all have their own unique use cases, so no matter what a company's security maturity is, the CISO and the security team can feel confident leveraging a system's recommendations, knowing it makes these recommendations and decisions based on microanalysis that goes far beyond the scale any single individual or team can expect of a human in the hours they have available. In this way, organizations of any type and size, with any use case or business need, will be able to leverage AI decision-making in a way that suits them, while autonomously detecting and responding to cyberattacks and preventing the disruption they cause.
About the Author
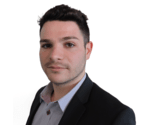
As VP of Product at Darktrace, Dan Fein has helped customers quickly achieve a complete and granular understanding of Darktrace's product suite. Dan has a particular focus on Darktrace email, ensuring that it is effectively deployed in complex digital environments, and works closely with the development, marketing, sales, and technical teams. Dan holds a bachelor's degree in computer science from New York University.
You May Also Like