AI Is Everywhere, but Don't Ignore the Basics
Artificial intelligence is no substitute for common sense, and it works best in combination with conventional cybersecurity technology. Here are the basic requirements and best practices you need to know.
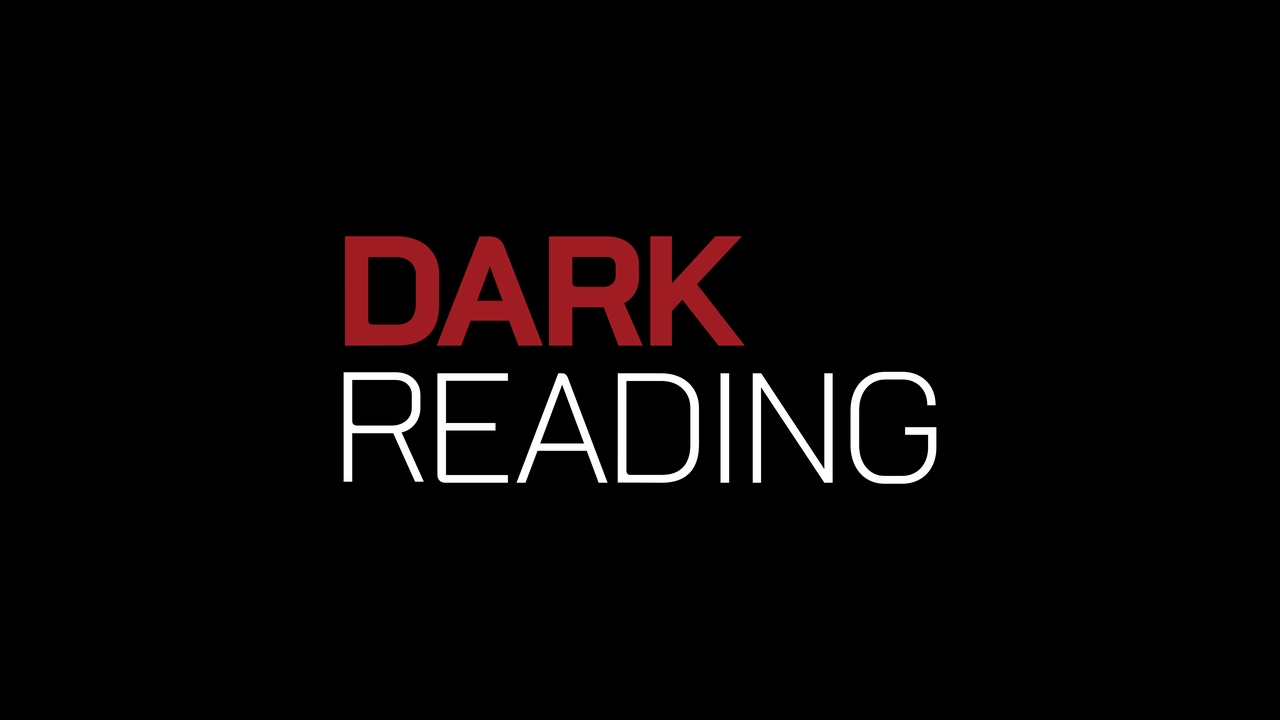
The fourth industrial revolution is here, and experts anticipate organizations will continue to embrace artificial intelligence (AI) and machine learning (ML) technologies. A forecast by IDC indicates spending on AI/ML will reach $35.8 billion this year and hit $79.2 billion by 2022. Though the principles of the technology have been around for decades, the more recent mass adoption of cloud computing and the flood of big data has made the concept a reality.
The result? Companies based around software-as-a-service are best positioned to take advantage of AI/ML because cloud and data are second nature to them.
In the past five years alone, AI/ML went from technology that showed lots of promise to one that delivers on that promise because of the convergence of easy access to inexpensive cloud computing and the integration of large data sets. AI and ML have already begun to see acceleration for cybersecurity uses. Dealing with mountains of data that only continues to grow, machines that analyze data bring immense value to security teams: They can operate 24/7 and humans can't.
For your cybersecurity team to effectively launch AI/ML, be sure these three requirements are in place:
1. Data: If AI/ML is a rocket, data is the fuel. AI/ML requires massive amounts of data to help it train models that can do classifications and predictions with high accuracy. Generally, the more data that goes through the AI/ML system, the better the outcome.
2. Data science and data engineering: Data scientists and data engineers must be able to understand the data, sanitize it, extract it, transform it, load it, choose the right models and right features, engineer the features appropriately, measure the model appropriately, and update the model whenever needed.
3. Domain experts: They play an essential role in constructing an organization's dataset, identifying what is good and what is bad and providing insights into how this determination was made. This is often the aspect that gets overlooked when it comes to AI/ML.
Once you have these three requirements, the engineering and analytics teams can move to solving very specific problems. Here are three categories, for example:
1. Security user risk analysis: Just like a credit score, you can come up with a risk score based on a user behavior — and with AI/ML, you can now scale it for a very large-scale users.
2. Data exfiltration: With AI/ML, you'll be able to identify patterns more readily — what's normal, what's abnormal.
3. Content classification: Variants on web pages, ransomware strains, destination, and more.
Adopting AI/ML in your cybersecurity measures requires you to think differently, plan and pace the project differently, but it doesn't replace common sense and some of the conventional best practices. AI/ML is not a substitute for having a layered security defense, either. In fact, we've seen that AI/ML has been doing far better when combined with traditional cybersecurity technology.
Here are three tenets to execute an AI/ML project:
1. "Not all data can be treated equal." Enterprise data has custom privacy and access control requirements; the data often is spread around different departments and encoded with a long history of "tribal knowledge."
2. "Wars have been won or lost primarily because of logistics," as noted by General Eisenhower. In the context of the AI/ML battleground, the logistics is the data and model pipeline. Without an automated and flexible data and model pipeline, you may win one battle here and there but will likely lose the war.
3. "It takes a village" to raise a successful AI/ML project. Data scientists need to have tight alignment with domain experts, data engineers, and businesspeople.
In the past, there have been two main criticisms of AI/ML: 1) AI is a black box, so it's hard for security practitioners to explain the results, and 2) AI/ML has too many false positives (that is, false alarms). But by combining AI/ML and tried-and-true conventional cybersecurity technology, AI/ML is more explainable, and you get fewer false positives than with conventional technology alone.
AI/ML already proved it can help businesses in a number of ways, but it still lacks context, common sense, and human awareness. That's the next step toward perfecting the technology. In the meantime, cybersecurity defense still requires domain experts, but now these experts are helping shape the future with a new paradigm shift for AI/ML methodology.
Related Content:
Check out The Edge, Dark Reading's new section for features, threat data, and in-depth perspectives. Today's top story: "Phishers' Latest Tricks for Reeling in New Victims."
About the Author
You May Also Like