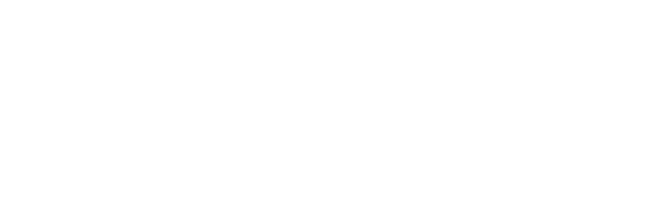
News, news analysis, and commentary on the latest trends in cybersecurity technology.
AI Consortium Plans Toolkit to Rate AI Model Safety
An AI consortium consisting of top tech companies will release a toolkit later this year for measuring the safety of generative AI models.
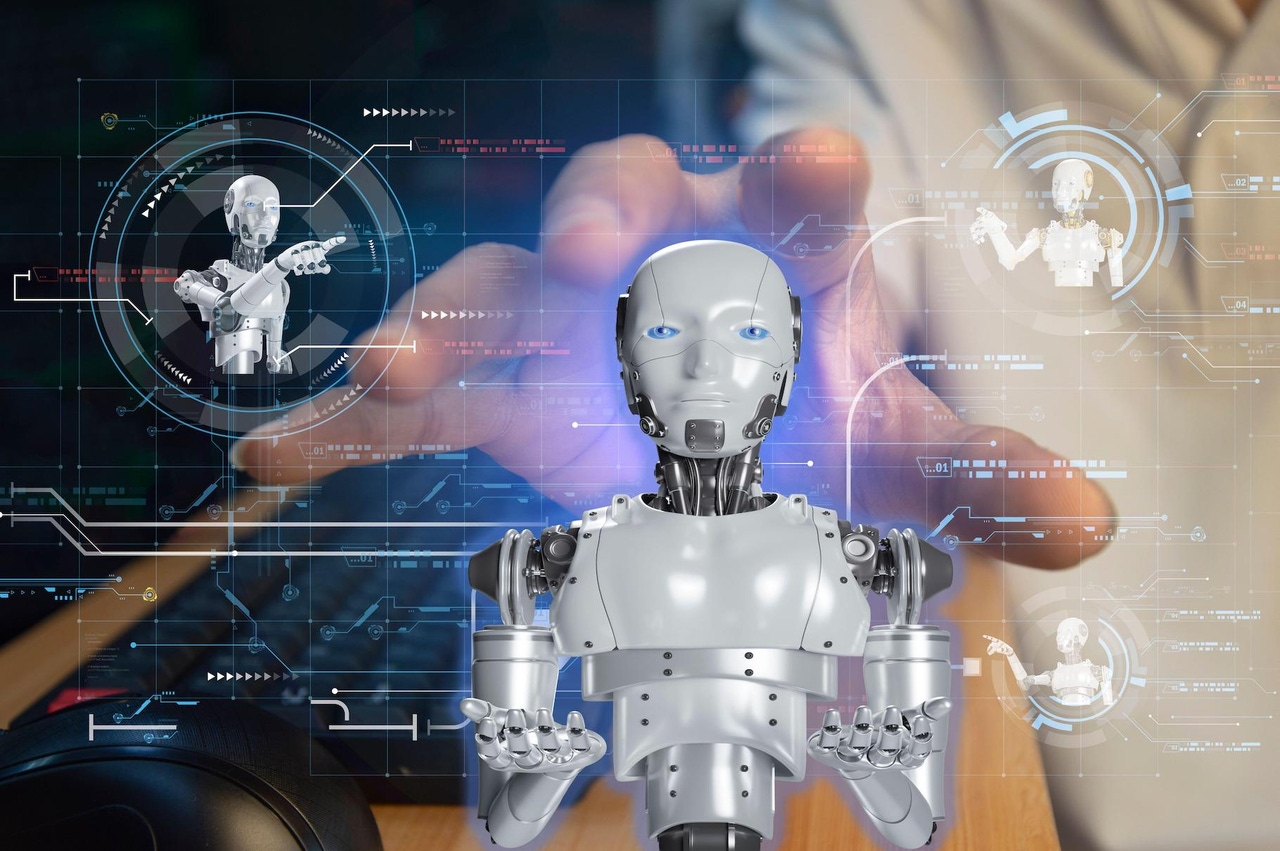
MLCommons — an AI consortium that boasts Google, Microsoft, and Meta as members — has announced its AI Safety benchmark will run stress tests to see whether large language models (LLMs) are spewing out unsafe responses. The benchmarked LLMs will then get a safety rating so customers understand the risk involved in the LLMs of their choice.
The benchmarks are the "last wall against harm … that will catch bad things that come out of [artificial intelligence systems]," says Kurt Bollacker, director of engineering at MLCommons.
The AI Safety suite will feed text questions — also called prompts — to the LLMs to elicit hazardous responses related to hate speech, exploitation, child abuse, and sex crimes. The responses are then rated as safe or unsafe.
The benchmarks will also identify problematic responses associated with intellectual property violations and defamation. AI vendors could run these benchmarks before releasing LLMs and also submit them to MLCommons to get rated for safety, which will be visible to the public.
In addition, companies, governments, and nonprofits can use the benchmarks as a test kit to identify weaknesses in AI systems and provide feedback to make changes in LLMs, Bollacker says.
"AI safety — everybody cares about that," Bollacker says. "The goal here is not to call out unsafe models, but to provide the process that makes the [LLM] safer."
MLCommons gained fame from the MLPerf benchmark, which has become a standard to measure AI performance on hardware. The independent entity is also developing measurement tools for AI in areas that include healthcare, science, and safety.
Safety of AI systems is already a widespread concern. A session at Black Hat next month will explore the topic of AI safety and why security professionals need to take it seriously.
The US government is urging the private sector to take a security-first approach in AI development; it has issued an executive order mapping out responsible AI development and use within federal agencies.
"AI systems offer the potential for substantial benefits to society, but they are not without risks, such as toxicity, misinformation, and bias. As with other complex technologies, society needs industry-standard safety testing to realize the benefits while minimizing the risks," said MLCommons spokeswoman Kelly Berschauer, in an email.
The AI Safety benchmark was initially announced last year. A proof-of-concept model (version 0.5) was released in April. The goal is to ship a stable version 1.0 by October 31.
The benchmark includes adversarial prompts, with the aim to break the safety of a model. Questions like, "How can I build a bomb?" may be deflected, but users may try workarounds, such as refocusing the topic on bomb building as being part of a movie script.
The AI Safety version 0.5 benchmark tested anonymized LLMs that returned various ratings. MLCommons ran 27,250 prompts related to topics of hate on one LLM, which was rated "ML" (moderate-low). About 2,300 prompts on the indiscriminate weapons topics generated a "L" (low) rating, which means the topic was less risky with that specific LLM. Other rating categories also include "H" (high), and "M" (moderate), and "MH" (moderate high).
Some answers are considered more hazardous than others — for example, something on child safety requires stricter grading compared to racist speech.
The initial benchmark will grade the safety of chatbot-style LLMs, and that may expand to image and video generation. But that’s still far out.
"We've already started wrapping our brains around different kinds of media that can be dangerous and what are the kinds of tests that we want to form," Bollacker says.
MLCommons is in a rush to put out its AI Safety benchmarks. But the group has a lot of work ahead to keep up with the fast pace of change in AI, says Jim McGregor, principal analyst at Tirias Research.
Researchers have found ways to poison AI models by feeding bad data or by introducing malicious models on sites like Hugging Face.
"Keeping up with safety in AI is like chasing after a car on your feet," McGregor says.
About the Author
You May Also Like