Building A Detection Strategy With The Right Metrics
The tools used in detecting intrusions can lead to an overwhelming number of alerts, but they’re a vital part of security.
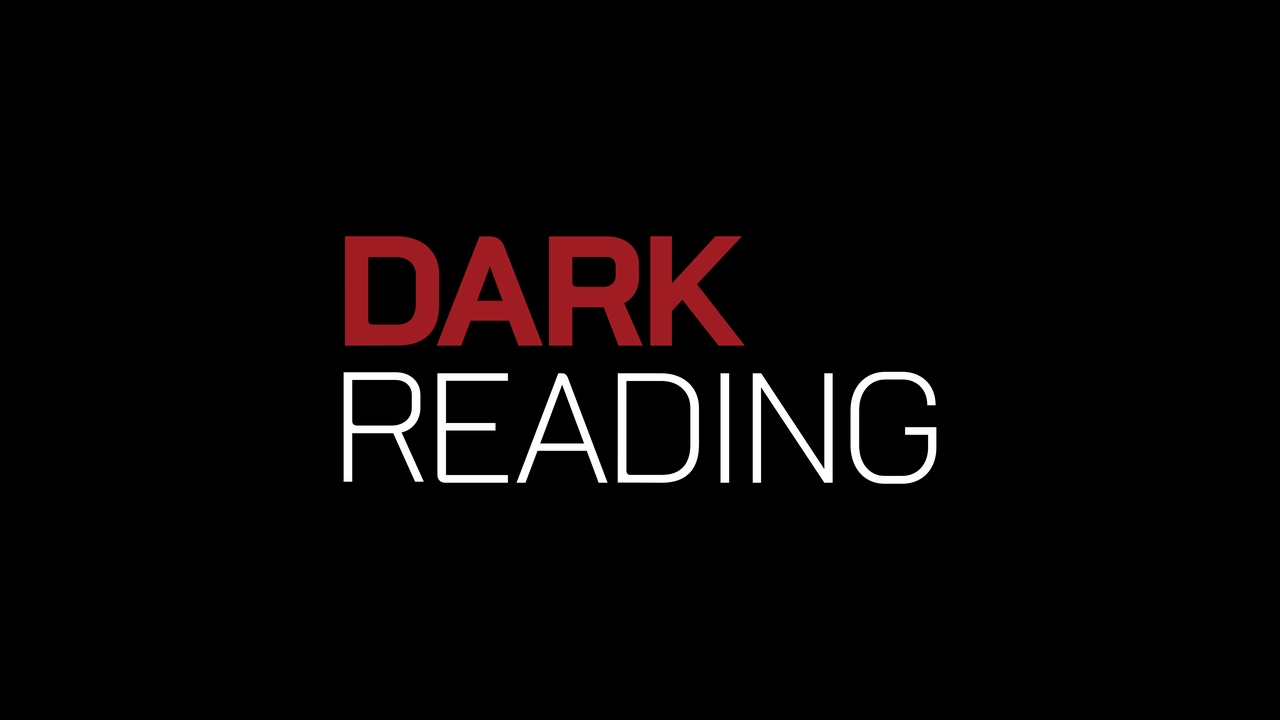
Traditional security used to be focused exclusively on prevention and policy enforcement. While these mechanisms are still important, there is increasing attention paid to detection in order to be able to more rapidly spot potentially malicious activities that have circumvented preventative security.
The leading security teams are shifting their mission to detect a potential attack and respond appropriately and quickly. To achieve such a mission, organizations typically implement the following detection (or incident response) process:
Start with an alert.
Investigate the alert and determine if it is benign or unwanted.
Make a proper decision and remediate or respond.
This detection process can be implemented at different levels of detail in various security organizations. I have seen implementations conducted by a single-person security team all the way to large security operations centers.
Of course, an effective detection strategy isn’t easy to achieve with a limited security budget and constrained staff. A major factor determining the effectiveness of the detection process is that security teams have to deal with the massive inefficiency and imprecision of security tools. For most organizations, the total alert volume is far beyond what any group can handle. Most alerts aren’t useful because either they don’t highlight anything of importance or are nonspecific or unsubstantiated, making an already impossible situation even worse. This situation is created by three main factors.
First, most tools are still primarily malware-oriented and based on technical artifacts, such as a signature, hash, domain, or a predefined list of software behaviors. This means that alerts will be firing all the time.
Second, many tools are designed to detect isolated “trees” and don’t understand that they are part of an overall “forest” of an orchestrated campaign being run by an attacker. It’s common to see a singular event — let’s say a scan inside the network — set off a hundreds or even thousands of individual alerts.
Third, many traditional tools are based on a do-it-yourself mentality. They’re created assuming a completely manual style of operational security. Part of this recognizes that most tools are rather low in fidelity and not intended to offload the work of a security professional by conducting some level of investigation or making a judgment call.
If organizations are going to be successful with detection, they need to measure and improve their efficiency. The following questions can help develop an effective detection strategy, or evaluate a specific new or existing detection tool:
Detection coverage: If an active attacker were operating inside my network, would my systems see an operational activity and set off an alert?
Detection quantity: Can my team investigate all the relevant alerts? Is it clear which are relevant?
Detection quality: When an alert is investigated, can I reach a conclusion?
Detection coverage is determined by what is being sought — is a tool looking for malware? Reconnaissance? Lateral movement? The best approach here is to define a few use cases that you’d like to detect and make sure that they’re covered. Most data breaches are ultimately the result of an attacker that operates inside the internal network. The use cases can either be defined theoretically or tested live as an attack simulation or penetration test.
Detection quality and quantity can be measured in a very concrete way using standardized metrics:
Detection quantity: efficiency — Count the total number of alerts per day and calculate how many alerts you have per 1,000 hosts.
Detection quality: accuracy — Percentage of alerts handled by the team that are useful (not whitelisted or ignored) over the total number of alerts.
A Ponemon Institute study of 630 enterprises showed that the average number of alerts a security team receives per week from their security tools — firewalls, intrusion-detection systems, intrusion-prevention systems, sandboxing systems, security information and event management (SIEM) systems, etc. — was a shocking 172 per 1,000 hosts per day (2,420 alerts for an average 14,000 endpoints in a network). Where does one start with that volume of alerts, particularly given that the large majority of these are false-positives? The same study shows that, on average, two-thirds of the security staff’s time is wasted because their tools are grossly inefficient, so only 4% of alerts are typically investigated, leaving the possibility that somewhere around 96% of those untouched may be something important.
This is clearly an inefficient, inaccurate process because there is no good way to guarantee a focus on the right alerts. There are examples where signs of an attacker were hidden in a flood of alerts. In the Target breach, according to reported details, signals were detected by existing systems but buried under thousands of similar alerts without any way to know that this particular alert was important.
It’s not enough to have a haystack with some needles that can only be discovered with hindsight after it’s too late; we need a process that can get to the important needles to proactively detect attacks, before damage is done. Realistically, security teams can actively handle a handful of alerts per 1,000 hosts per day. This is based on the Ponemon data (4% of handled events equals 6.8 alerts/1,000 hosts/day) and also on my experience with different chief information security officers. If a process generates many more, they will be ignored.
Using metrics enables evaluation and comparison of different tools and methods, including overlapping products. For example, you can compare SIEM-based event correlation to network traffic analytics, sandbox-based detection, user and entity behavior analytics, endpoint detection, malware analysis, and so on, with these questions:
Does the type of new alerts add significant coverage or attack detection capability?
How many alerts per day does the system normally generate? Can my team investigate that amount?
What percentage of the alerts can be handled and are relevant to handle?
With this perspective, we can ask questions such as, “Will this increase detection accuracy?” “How is this security tool making the team more efficient?” and “Can this security solution reduce staffing requirements?”
Related Content:
About the Author
You May Also Like