How Machine Learning For Behavior Analytics & Anomaly Detection Speeds MitigationHow Machine Learning For Behavior Analytics & Anomaly Detection Speeds Mitigation
By relying on artificial intelligence to identify suspicious network activity or behavior, machine learning can adapt to both business needs and new threats.
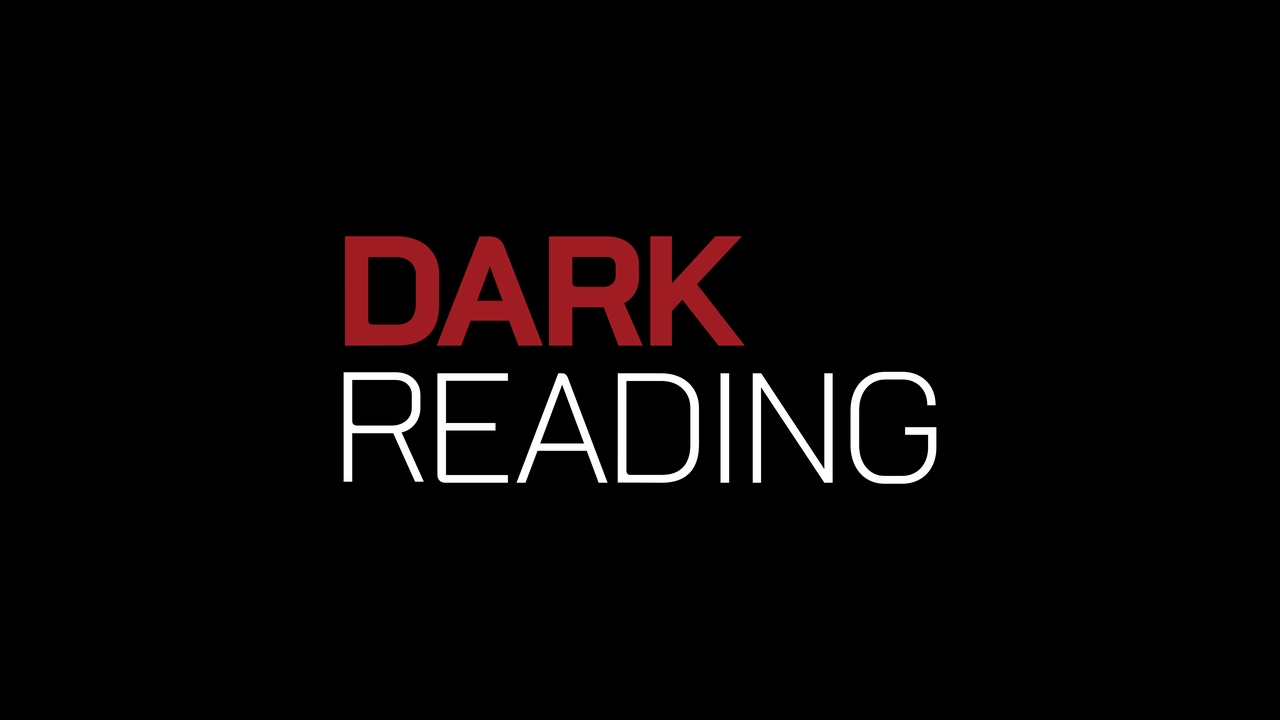
Businesses and organizations are under heavier fire than usual from cyberattacks, with 57% of CIOs and CISOs reporting at least one significant cybersecurity incident at their companies. Whether the attacks resulted from unaware employees (55%), unauthorized access (54%), or malware (52%), security decision-makers have opted to increase their security budgets to adopt new technologies and cybersecurity defenses.
Business-centric machine learning for behavior analytics and anomaly detection should be adopted by any organization focused on faster detection and mitigation to prevent advanced persistent threats (APTs) from significantly impacting their business. By relying on artificial intelligence to identify suspicious network activity or behavior, machine learning can adapt to both business needs and new threats.
Bitdefender has been developing and using patented machine-learning algorithms since 2009, constantly tweaking and improving them to proactively detect new and never-before-seen malware.
Your Enterprise Network Is Predictable
Starting from the premise that your enterprise network is predictable, deploying behavior analytics technologies requires first observing and learning your organization’s network behavior. Afterward, anything new or out of the ordinary that doesn’t respect the learned behavior will be reported to IT managers.
However, it’s important to note that you can use these technologies for either spotting new processes that are suspicious for that network, or spotting behavior that’s abnormal. For example, after training, machine learning can create a prediction database that will contain all known applications currently deployed in your organization.
What happens to the prediction database when a company‘s deployed application is updated, after the training process is completed? That’s when the adaptation on variation to the baseline kicks in and machine learning flexes its muscles. When the updated application runs for the first time, the machine-learning detection module checks if the prediction database contains the launched application. If a perfect match isn’t found, it will apply a similarity factor that statistically estimates the chances for the unknown application to be similar to something the database already has. If that similarity percentage passes a specific threshold, the application is considered trusted and the prediction database is updated. If the similarity score is below the threshold, the application is quarantined and the IT administrator is notified.
Application Profiling with Machine Learning
Profiling applications with machine learning requires the use of various algorithms such as binary decision trees, neural networks, and genetic algorithms, but it all starts with building a model that can be used for accurate detection. Because a model is actually an automatically generated mathematical equation that satisfies a set of conditions known to be associated with a malicious file, its purpose is to statistically estimate the chances that an unknown or never-before-seen file is malicious.
Neural networks are among the most commonly used types of machine-learning algorithms, as they can extract file characteristics into features -- file form, emulator information, and compiler type, among others -- and normalize those features into numbers. Of course, not all features are used to train a model, but just a subset of them can actually yield highly accurate results. All these features are placed in N-dimensional matrixes, where N represents the number of features, and then they generate highly complex equations (or models) that accurately identify unknown samples as malicious or not, based on whether the equation is met.
Put another way, if an unknown file reaches an organization’s perimeter and ends up being fed into a machine-learning algorithm that uses such models, the file is tested on whether it resolves a series of mathematical equations known to be resolved only by malicious files or applications.
Is Machine Learning Reliable in Business Environments?
If the average user displays an unpredictable behavior in his or her online and PC activities, the business environment -- from network traffic to endpoint activity -- is pretty much predictable, and therefore a baseline can be performed. Machine learning can sniff through large amounts of data and make an “educated” -- or statistically accurate -- guess on whether something abnormal is going on.
While training the machine model may take some time, the resulted expression (or equation, as previously referred to) is usually just a couple of kilobytes in size, meaning that it’s really fast to compute and has a very low memory footprint. Naturally, having more models specifically trained to analyze specific behaviors is always recommended, as they can cover a wide array of potential attack vectors, warning security teams of impending and potential security threats.
The merging of human and machine learning is vital in training accurate machine-learning models, and organizations have a lot more to gain by working with technology security companies that have been actively involved in machine-learning development for years.
About the Author
You May Also Like