Improving Attribution & Malware Identification With Machine Learning
New technique may be able to predict not only whether unfamiliar, unknown code is malicious, but also what family it is and who it came from.
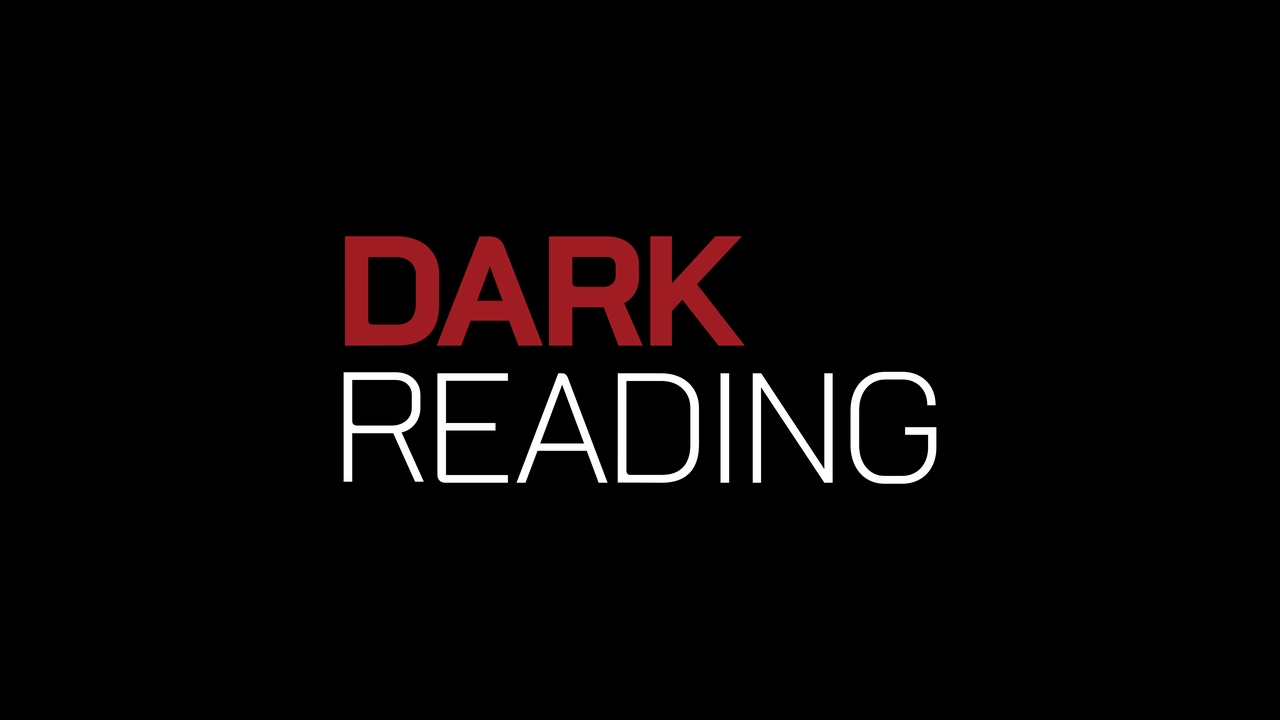
One of the cybersecurity promises of machine learning (particularly "deep learning") is that it can accurately identify malware nobody has ever seen before because of what it's learned about malware it's seen in the past. Konstantin Berlin, senior research engineer at Invincea Labs, is trying to take the techology further, so that organizations can get more information about unfamiliar code than simply "it's benign" or "it's malicious."
Berlin, who will be presenting his work next month at Black Hat, says security pros also want to know more about the malware family so they can plan their mitigation strategy accordingly. His technique, he says will do that, as well as improve malware triage and attribution by using new methods of recognizing similarities between malware samples. This can all be done in a customized way that enables each organization to choose what features and factors interest them most.
Berlin explains machine learning's difference to traditional signature-based anti-malware like this: If, for example, you want to predict the direction a rocket will go when it sets off, he says you don't necessarily need to learn the physics of propulsion and enter equations into the machine. You simply need to feed it lots of data of examples of rockets going off until it learns to accurately predict where the rockets will go. "Based upon millions of observations, it won't necessarily explain the rule, but it works in terms of prediction."
So, even if the machine has never seen something before, it will know it's malicious -- even if it doesn't know precisely why.
What Berlin wants to do, however, is give people more than just benign or malicious.
To do that, he's using a technique that improves the way security tools recognize what binary is similar to another -- and therefore how they are classified into families, attributed to malware authors, and tied to threat actors.
According to Berlin, the current process usually used is expensive to develop, and requires periodic retuning that is done manually because organizations have their own sets of features they look for in malware binaries, their own weighting system for which features are most significant, and their own methods for minimizing the impact of those features that aren't important at all. Because of the costs and the labor, the retuning isn't done as often, and therefore it's more difficult to keep up with the pace of malware evolution.
The method Berlin is presenting at Black Hat next month may not only improve accuracy but make the process cheaper, he believes. It uses a technique called supervised embedding, and is something the security world more commonly encounters in facial recognition.
Supervised embedding is a way to disregard malware samples' unimportant features, enhance their most important features, and re-map the distance between those malware samples. Distance thus mirrors "semantic sense" and similarity is measured by the features the security team has deemed are the most essential for their needs. So, if they're specifically interested in principally grouping malware by the likely threat actor, target industry, attack vector or attack type, they could. Any features of a file that are unrelated to whether it is malicious are automatically eliminated, says Berlin, "so the distances rely on the tradecraft of the malware."
It does not require a stack of signatures, but the technology does require a database of labels for all of these malware features. Berlin is using Microsoft's existing database of families and variants, but organizations could invest in creating their own bespoke database that truly zeroes in on the information they want.
"That's the beauty of machine learning," he says. "You train it for the task you want to accomplish."
This sort of system, this brain, is considerably lighter to carry around than a stack of signatures, too, says Berlin. This "statistical approach," requires less power than an "all or nothing" approach, he says.
Related Content:
Black Hat USA returns to the fabulous Mandalay Bay in Las Vegas, Nevada July 30 through Aug. 4, 2016. Click for information on the conference schedule and to register.
Read more about:
Black Hat NewsAbout the Author
You May Also Like