Machine Learning Susses Out Social-Network Fraud
Machine-learning techniques can be used to detect fraud and spies on social networks based on certain features, such as the number of followers and devices used to access the network
April 19, 2013
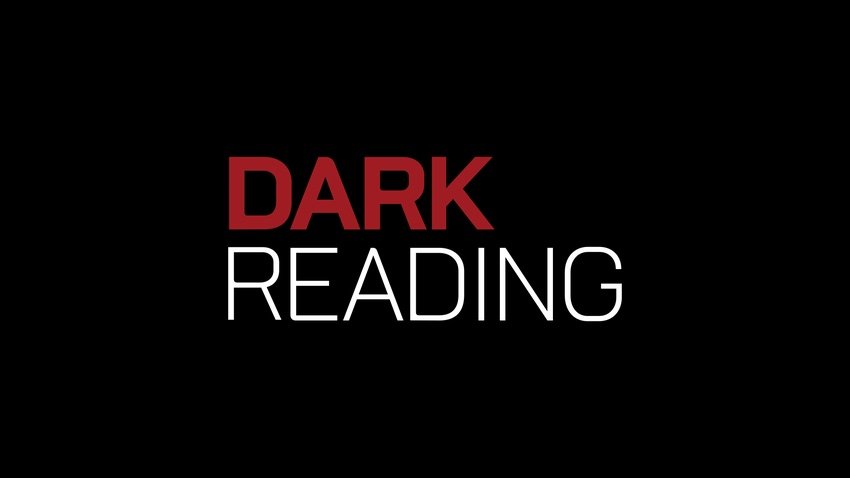
Certain characteristics of social-network accounts have a high correlation with fraud and can be used to differentiate between real and fake accounts, a researcher presenting at the SOURCE Boston Conference said this week.
Using machine-learning techniques, Vicente Diaz, a senior security analyst with security software firm Kaspersky Lab, found that seven characteristics of Twitter profiles could identify fraudulent accounts 91 percent of the time. The number of devices from which a user accesses the service, the ratio of followers to people following an account, the average number of tweets to each person, and the number of tweets to an unknown receiver are all features that correlate strongly to fraudulent accounts, he says.
"Surprisingly, it was quite easy to identify the malicious profiles," Diaz says. "The most important thing for you to keep in mind is to select a smart set of features."
As social networks have become more popular, the questionable uses of the highly connected social circles has rapidly grown. Twitter, Facebook, and other networks are increasingly used for spam as a way to gather information on users and to distribute malware. Fake accounts controlled by a single user are an essential part of the fraud schemes. In many cases, the accounts are created, built up, and then harvested and sold off, says Chris Porter, principal with Verizon's RISK group.
"They are trying to amass a lot of followers, and then they will sell the account off to other parties," Porter says. "Organized crime could use that information to pump links to potential victims to spread malware or other schemes."
Last year, Facebook estimated that almost 9 percent of the accounts on its network are fraudulent. One security firm, Impermium, has put the number much higher: As many as [Using the social context of posts, researchers from UC Riverside create prototype Facebook app that detects social malware with 97 percent accuracy. See Application Detects Social Network Spam, Malware.]
Yet while spam and fraud are a problem, malware is far less of an issue, according to Palo Alto Networks, a network security provider. In a recent report, the company found that while 20 percent of average traffic on the network is due to social networks, malicious traffic emanating from social networks accounted for less than 0.2 percent of all threat logs.
"Facebook and Twitter have some pretty good security teams in house," says Michael Sutton, vice president of research for Web-security firm Zscaler. "Most of the scams that we see have a shelf life of about an hour. They are doing a good job."
Kaspersky's Diaz agreed. By looking at almost 13,500 profiles with 6.5 million relationships, the researcher found that most fake accounts were engaged in fraud -- not malicious attacks. The researchers used a number of machine learning techniques, such as radial-basis function (RBF) neural networks and Bayesian filtering, to recognize patterns, including 36 malicious campaigns.
In addition, he found that he could identify hacked accounts with an 80 percent accuracy rate. By monitoring accounts using a small time window, Diaz's system could detect changes in behavior that indicated an account had become compromised.
To continue his research, Diaz wants to apply his models to the large population of accounts that follow corporations and celebrities, trying to find fake accounts and understand how fraudsters use the popular crowd to fuel their own schemes.
"It will be interesting to see how many followers are real and how many are fake profiles used to boost the apparent fans of a celebrity," Diaz says.
Have a comment on this story? Please click "Add Your Comment" below. If you'd like to contact Dark Reading's editors directly, send us a message.
About the Author(s)
You May Also Like
Why Effective Asset Management is Critical to Enterprise Cybersecurity
May 21, 2024Finding Your Way on the Path to Zero Trust
May 22, 2024Extending Access Management: Securing Access for all Identities, Devices, and Applications
June 4, 2024Assessing Software Supply Chain Risk
June 6, 2024Preventing Attackers From Wandering Through Your Enterprise Infrastructure
June 19, 2024
Black Hat USA - August 3-8 - Learn More
August 3, 2024Cybersecurity's Hottest New Technologies: What You Need To Know
March 21, 2024