Myth-Busting Machine Learning In Security
Black Hat USA presentation to help quell misconceptions and confusion over machine learning methods in today's security tools.
July 28, 2014
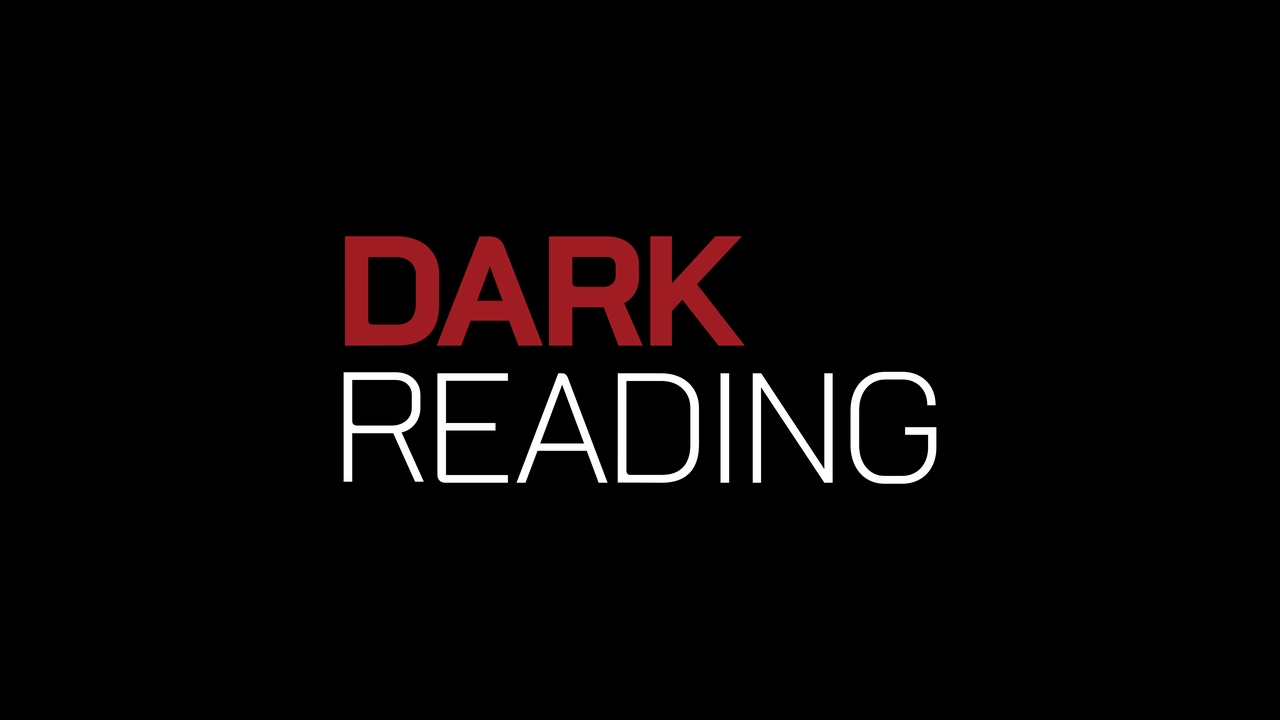
As increasingly more security tools are touted today as being backed by big data, anomaly detection, behavioral analysis, and algorithmic technology, security practitioners should be wary. According to a talk slated for Black Hat USA next week, the interest has grown among security rank and file to employ machine learning to improve how they solve tough security problems.
But it's mostly the marketing arms of vendors that have caught up to this interest -- not the actual technology.
"Don't get me wrong, there are techniques that work if applied correctly," says Alex Pinto, a former security consultant and founder of the MLSec Project, a year-old research project dedicated to investigating machine learning techniques using live data from real enterprises. "But most of the claims I am seeing are rebranded old techniques."
As Pinto explains, most of the tools marketed as using advanced algorithmic analysis of behavior or anomaly detection has actually been around in tools in the same exact form for 10 years and around in research for 30 years. And some of the mathematically based capabilities that vendors are claiming to have cracked are actually very hard "open" problems in the theoretical world.
"When you think about true anomaly detection or behavior analysis, the challenge is that security is grasping at straws because it wants the algorithm to figure out if something is normal or not," says Pinto, who will lead a talk called "Secure Because Math: A Deep Dive On Machine Learning-Based Monitoring at Black Hat." "That works well if you're only measuring one variable. But if you increase that and try to analyze, say, the NetFlow of 1,000 different machines talking to each other, today's theoretical mathematical capabilities have no chance."
If they did, they would be in use offering breakthroughs in DNA analysis to figure out if certain people would be susceptible to specific diseases, or more lucratively, to further drive data-driven marketing campaigns, because the underlying mathematical problems are similar. His goal with the talk is to show security people, who may not have a ton of theoretical math background, some of the right lines for grilling vendors when they come knocking with claims of advanced algorithms but aren't being very transparent about what is actually under the hood.
"If people are not able to answer these questions, they either don't know what they're doing or they're just point-blank lying about using machine learning," Pinto tells us.
He says he has three main things to warn buyers to look out for. The first is understanding where the information for the machine learning models is coming from.
"If it comes all from your environment, it could be susceptible to tampering by attackers. Also not having strictly identified what are the events that should be singled out by the models makes them very fragile. Pure and simple anomaly detection usually falls into this dangerous area, and these tools end up being very prone to false positives."
The second is asking about the underlying assumptions of the models. One of the big pitfalls of user behavior analysis and leak detection technology is the big assumption that the buying organization already has a very good information classification labeling of its data and solid hierarchical definitions of the users' roles. He warns that most corporate organizations have a long road ahead to establish that. Finally, the third question to be answered is how the technology is meant to be used and how it integrates with current security processes.
"Even the best models have some degree of false positives, and you need support from your organization to manage and handle this. This is again at odds with the life-saving and miraculous way machine learning is portrayed. You know Facebook ads? They get stuff wrong all the time. It is the same tech! Sometimes the ML might just get in the way of your processes."
Pinto says that he came up with the topic idea almost in direct opposition of his talk last year, which was meant to pump up interest in machine learning's potential to improve security. He's still a big believer in machine learning, but he wants to bring the hype down a bit so that scurrilous marketing doesn't give the entire field of machine learning a bad name. The idea is to get security practitioners to understand that just because a vendor claims to have PhD mathematicians on staff doesn't mean that it's magically solved security's problems.
"To be honest, I want to provoke vendors who are doing this to be more transparent."
In conjunction with his warnings, he'll also offer a silver lining in the form of an update on some of the research breakthroughs his team at MLSec has had in the past year.
Notably, he'll discuss how they're looking for better ways to use machine learning to interrogate threat intelligence feeds in order to fine tune and speed up analysis and point practitioners and operations staff in the right directions more quickly. As he explains, experienced security practitioners know that while some of these indicator feeds could be used as simple black lists, they're best used for experienced analysis. This human investigation usually involves learning patterns and intrinsic characteristics such as Internet routing positions or data centers hosting threats.
"These are very labor-intensive processes, and it turns out they can be efficiently mined for features for machine learning," says Pinto. "In a nutshell, our models are able to extrapolate the knowledge of existing threat intelligence feeds as experienced analysts would. When you run them against log data from one of our participants, their data helps fill out some knowledge gaps and biases the model may develop during the training process and returns a very short list of potential compromised machines."
He says to think of it like an Amazon recommendation system for network security:
" 'Your peers have just been hacked like this -- you may want to look at these guys on your network.' Of course, it is not magical, and some few false positives creep up now and then, but the organizations working with us have been very receptive to the results."
Read more about:
Black Hat NewsAbout the Author
You May Also Like