Deepfakes & James Bond Research Project: Cool but Dangerous
Open source software for creating deepfakes is getting better and better, to the chagrin of researchers
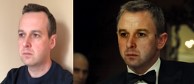
In January 2020, NCC Group collaborated with University College London (UCL) students on the topic of cybersecurity implications of deepfakes. As part of our wider research into artificial intelligence (AI) and machine learning, we continue to explore the potential impact of deepfakes in a cybersecurity context, particularly around their use in nefarious activities. There have already been numerous stories of real-world fraudsters using AI to mimic CEO voices in cybercriminal activities, and we believe it's only a matter of time before we see similar, visual-based attempts using deepfake frameworks. And remember, many of those frameworks are open source and freely available for experimentation.
Project & Challenge
Our brief to the students (who are part of UCL's Centre for Doctoral Training in Data Intensive Science) was to explore common open source deepfake frameworks and broadly assess them in terms of ease of use and quality of faked outputs. This first part of the research was to help us understand how accessible these frameworks are to potential fraudsters, and the computational resources and execution times needed to produce realistic outputs. We examined two in particular, FaceSwap and DeepFaceLab, and one open source speech-driven facial synthesis model.
We also asked them to help us explore the practicalities — specifically, how realistic fake videos can be achieved. The challenge was to take a three-minute clip from a movie (Casino Royale) and replace the face of the lead character (Daniel Craig playing James Bond) with my face. This helped us understand logistical aspects around source and destination video qualities, lighting conditions, angles, and facial expressions of source and target imagery. We also got a better understanding of not only the technical details but also the procedural and physical aspects.
On the procedural front, we learned that when trying to create realistic deepfakes, the quality (resolution) of source and destination image sets is very important, in that they should match very well. We lost some realism in the output because the initial HD quality source footage didn't match the cinematic effect of the target video, which had a smoother resolution. Lighting conditions are also important, and both source and target faces should be similar in shape. For example, our source image had to be slightly stretched to match that of the James Bond character.
Everyday objects also presented difficulties — the simple act of wearing glasses could make it easier to prevent deepfake attacks.
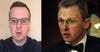
Procedurally, we also learned that it's harder to produce realistic deepfakes when the source image doesn't have the same types of mouth shape and movement (during dialogue) and eye movements related to raised eyebrows or blinking. Attackers seeking to create realistic deepfakes need a rich source facial image dataset of each individual with different facial expressions and angles.
What We Learned
Our research was designed to help us better understand technical risk mitigation strategies and/or policies, regulation, and legislation that might be needed to curb potential abuse of deepfake technology. Here's what we found:
There are many open source frameworks already available for creating deepfakes.
Many models are optimized for high-end PCs or HPCs, and require lengthy training.
The frameworks are easy to pick up but harder to master.
There is plenty of scope for human error, which results in unrealistic videos.
There are many procedural aspects that impede the creation of convincing deepfakes: lighting, angles, source, and destination faces of similar size and shape.
In terms of prevention, our research did identify a few existing techniques that offer varying degrees of deepfake detection. These largely rely on imperfections, which means that as models improve, the defensive measures will be less effective.
Preventative mechanisms pose an even bigger challenge: They require either the introduction of watermarking (which brings its own limitations) or the establishment of root of trust at the point of original content creation. These would be difficult to engineer and implement.
The prevalence of freely available and easy-to-use deepfake software is an ongoing concern. While there are still many procedural and computing roadblocks to creating realistic outputs, Moore's Law and history tell us it's only a matter of time before these technologies get better and more accessible. We need more research, more technology options, and perhaps regulation to help ward off deepfake dangers.
Related Content:
Register now for this year's fully virtual Black Hat USA, scheduled to take place August 1–6, and get more information about the event on the Black Hat website. Click for details on conference information and to register.
About the Author
You May Also Like