How AI Could Become the Firewall of 2003
An over-reliance on artificial intelligence and machine learning for the wrong uses will create unnecessary risks.
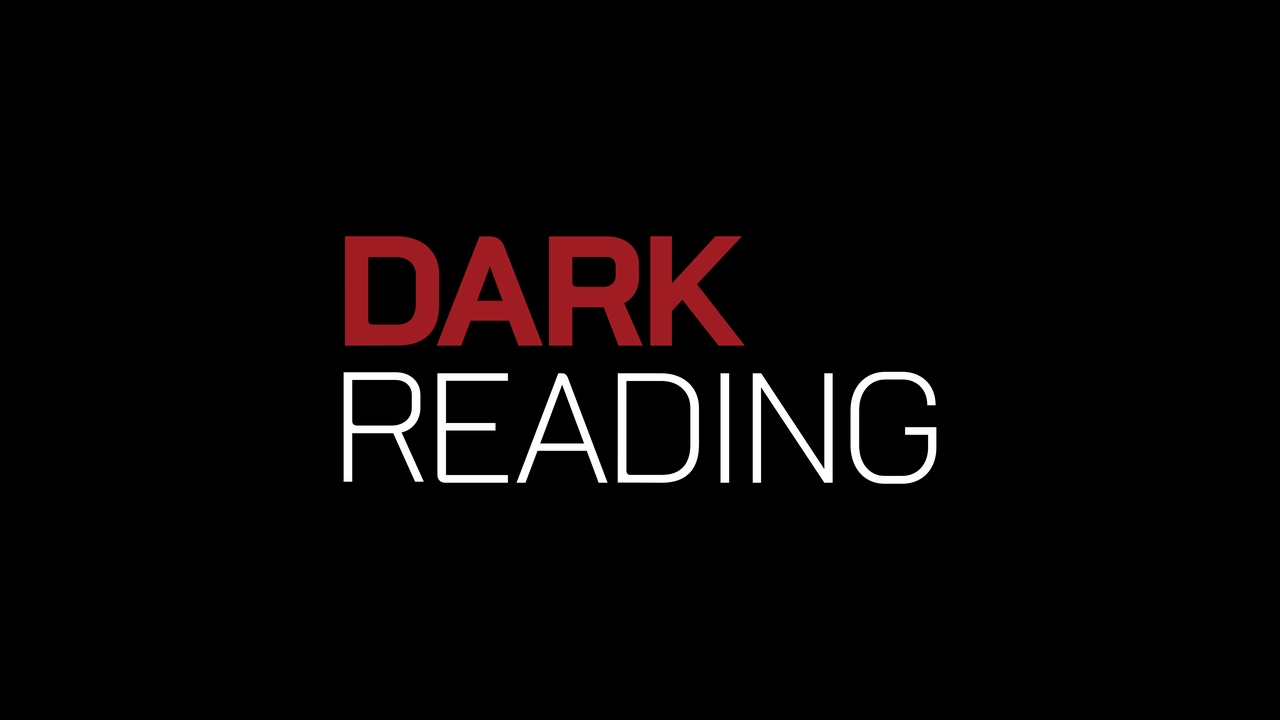
One of the shortcomings of the cybersecurity industry is a preoccupation with methodologies as solutions, rather than thinking about how they can be most useful. This scenario is happening right now with artificial intelligence (AI) and machine learning (ML) and reminds me of discussions I heard about firewalls back in 2003.
In 2003, pattern matching was the primary methodology for threat detection. As it became possible to perform pattern matching within hardware, the line between hardware-driven solutions (like firewalls) and software-based solutions — such as intrusion-detection systems — eroded.
Lost in this evolution was the fact that intrusion-detection systems went beyond pattern matching and included a combination of methodologies, including anomaly detection and event correlation, that never made it into firewalls. As a result, firewall-based pattern matching became the default solution for threat detection, as opposed to one important part of a whole.
This history is important because AI (really, ML) is simply another methodology in the evolution of tools that address specific aspects of the information security workflow.
Finding the Value of AI and ML in Security
Artificial intelligence is defined as having machines do "smart" or "intelligent" things on their own without human guidance. Machine learning is the practice of machines "learning" from data supplied by humans. Given these definitions, AI doesn't really exist in information security and won't for a long time.
ML solves a subset of well-defined security challenges far more effectively than existing methodologies.
Most of the time when you hear AI/ML referenced in marketing material, what's being described is heuristics, not computational statistics. Heuristics, while much simpler than AI, works very well on a variety of security activities while being far less computationally intensive than data science-based methodologies.
ML is simply one tool out of the methodology toolbox for identifying undesirable activity and is most successful in tackling well-bounded and understood problems.
Before I'm written off as simply a critic of AI/ML in security, I'll note that as one of the first employees at Cylance, I witnessed firsthand the stunningly successful application of ML to the problem of malware detection. However, that technical success was anchored in the boundedness of the problem being studied and solved. Specifically:
Structurally bound: The type of data and structure either don't change or slowly evolve over years. In this case, the structure of data is defined by file format specs.
Behaviorally bound: In a good use case for ML, the data being modeled will only appear as the result of a limited set of actions, allowing data points to be predictably mapped to understood behaviors.
Subversive-free influence: This is the most important factor and is almost exclusive to the context of information security. We face malicious humans who have an incentive to find and exploit weaknesses in the ML model. With that said, it's incredibly difficult to make enough changes to a file that obscure it from statistical analysis while remaining valid enough to load by an operating system.
Malware analysis and endpoint detection and response is one example of an infosec challenge that meets the three constraints above — which is why machine learning has been incredibly effective in this market.
Applying that same thought process to the network is dangerous because network data is not structurally or behaviorally bound and the attacker can send any sequence of 0s and 1s on the network. So does that mean AI and ML are a dead end for analyzing network data?
Yes and no. If the approach is to just use these powerful technologies to spot deviations from a baseline for every user or device, then we will fail miserably. The false positives and negatives produced by this "intelligent" approach will require a human to analyze the results before acting.
For instance, traffic analysis that alerts based on network anomalies may tell you there is too much traffic from an IP address that has never acted like this in the past. Often, the problem is that a new backup process is being rolled out. That puts us right back at the same skills crisis epidemic that AI was promised to solve.
Instead, what if we used AI and ML to determine good versus bad by comparing across the environment and specifically by comparing entity behaviors to those from entities that are most similar? This allows the system to automatically learn about changes such as the new backup process.
Not all infosec ML use cases are created equal — technically or philosophically. Like the firewall of 2003, machine learning does have some well-matched use cases that are advancing the state of the art in enterprise protection.
However, an over-reliance on machine learning for poorly matched use cases will burden the enterprise with unnecessary additional risk and expense while contributing to other lasting negative impacts such as the atrophying of methodologies that compensate for ML's weaknesses.
Related Content:
Learn from the industry's most knowledgeable CISOs and IT security experts in a setting that is conducive to interaction and conversation. Click for more info.
About the Author
You May Also Like