'Democratizing' Machine Learning for Fraud Prevention & Payments Intelligence
How fraud experts can fight cybercrime by 'downloading' their knowledge and experience into computer models.
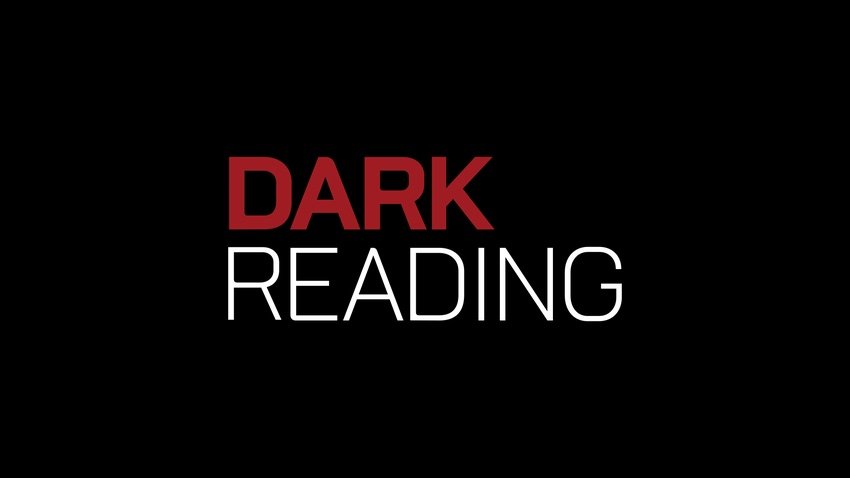
Throughout the financial industry, executives are acknowledging that machine learning can quickly and successfully process the vast volume and variety of data within a bank's operations — a task that's nearly impossible for humans to do at the same speed and scale. However, only about half of enterprises are using machine learning. Why? A bank-wide machine learning project is a huge undertaking, requiring major investment in technology and human resources.
Moreover, past projects that have failed to deliver a return on investment (ROI) have led to internal disappointment and, in some cases, mistrust in the technology. However, with smaller, more tactical machine learning projects that can be rapidly deployed, banks can reap the benefits from Day One. One such project is fraud prevention.
The current barrier to delivering fraud protection through machine learning often lies in the solutions themselves, which require data scientists to create the initial models. A fraud expert knows that a specific correlation between transaction types in a sequence is a strong fraud indicator, but the data scientist will need many more interactions with the same data to draw the same conclusion.
Fraud Experts or Data Scientists?
A machine learning model is only as good as the instructions it is given. This can be particularly challenging while setting up fraud prevention algorithms because fraud is a relatively small percentage of successful transactions, which means the model has fewer opportunities to learn. As a result, solutions that enable fraud experts, instead of data scientists, to input the initial correlations will deliver results faster in terms of identifying new correlations across different data sets as they're more familiar with the instances where fraud is likely to occur. This allows the organization to reduce the time to ROI of their machine learning projects.
This "democratization of machine learning" empowers fraud experts to "download" their knowledge and experience into computer models. It's particularly effective in areas where fraud has not yet reached critical mass to support fraud experts as they use their experience and instincts to investigate certain transactions or customer behaviors, even if they are not yet fraudulent or highly indicative of fraud. Feedback based on these kinds of instincts will aid the machine learning model to fine-tune itself, and improve accuracy and consistency in identifying more complex fraud indicators.
Teaching the Machine
Continuous involvement of fraud experts is key to developing the machine learning model over time. By bringing fraud experts closer to machine learning, they have transparent views into the models and can apply strategies and controls to best leverage the outcome of the intelligence from the models. As they input to the model, they're able to investigate the output as the model generates intelligence and use their human expertise to confirm fraud instances. They can also combine their all-encompassing customer view with insights correctly generated by the model.
If the "human intelligence" confirms the insights, these can be fed back into the model. If the model consistently flags a correlation between data sets as potential fraud and the analysts consistently confirm this as fraud, then a strategy and control based on this information can be added to underpin the model. These can, in turn, be used to automate the decisions that fraud analysts themselves have consistently made, and, ultimately, reduce the need for automatic actions that impact the customer experience, such as freezing a credit card when a suspicious purchase is made — even though the purchase is legitimate.
Related Content:
About the Author(s)
You May Also Like
Why Effective Asset Management is Critical to Enterprise Cybersecurity
May 21, 2024Finding Your Way on the Path to Zero Trust
May 22, 2024Extending Access Management: Securing Access for all Identities, Devices, and Applications
June 4, 2024Assessing Software Supply Chain Risk
June 6, 2024Preventing Attackers From Wandering Through Your Enterprise Infrastructure
June 19, 2024
Black Hat USA - August 3-8 - Learn More
August 3, 2024Cybersecurity's Hottest New Technologies: What You Need To Know
March 21, 2024