AIOps: The State of Full Packet Capture Enters the Age of Practicality
How machine learning and artificial intelligence are changing the game of acting on large volumes of network data in near real time.
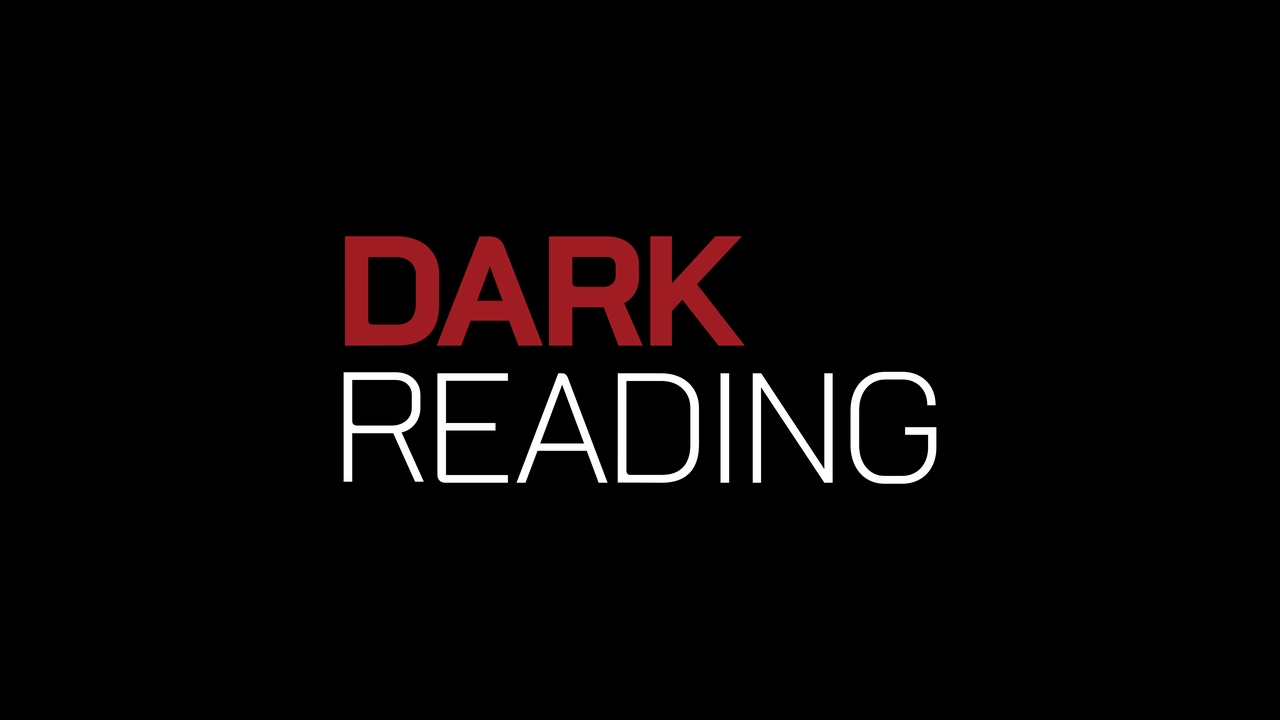
It's a great time to be a security analyst, but those who serve in the role today are facing much higher expectations from their organizations compared with when I started out. Many are teetering on the edge of burnout because their companies need to get to the truth sooner, leaving analysts stuck with traditional approaches and tactics associated with full packet capture as the high-speed network's bandwidth increases by the day.
The state of full packet capture — fundamental to enabling security analysts to hunt for threats, discover anomalies, or respond to incidents — has seen a few incremental advancements over the several decades but nothing that has allowed the analyst to allocate less time to it because there is still a bit of heavy lifting required.
As a security analyst in the military, my first experience with full packet capture in the late '90s was the SHADOW system, an open source project dubbed an intrusion-detection system but really a full packet capture system designed for retrospective analysis, also known as threat hunting. The project was essentially a framework built with tcpdump and a collection of Perl scripts. However, SHADOW lacked any form of indexing, so mining the data was quite painful.
The next breakthrough in full packet capture was Time Machine, which introduced the notion of connection cutoff and indexing for faster search and retrieval. A sister project to Zeek (formerly known as Bro), Time Machine was an interesting project with lots of promise. Unfortunately, Time Machine did not scale beyond a few gigabits per second. Finally, there is Moloch, a full packet capture and search application integrated with advanced visualization that scales to 10Gbps and more. Moloch represents the state-of-the-art in open source, full packet capture, but it is yet to be determined if it can scale to 100Gbps.
These incremental improvements were made in the background while the high-speed network expanded and has grown in importance within the organization. While the number of servers on-premises might have decreased, the quantity of mobile devices, Internet of Things sensors and cloud applications that organizations are utilizing today to improve operations is increasing to create an even more complex network environment, making the traditional approaches to full packet capture even more impractical.
Adding to the problem is the recent rise in overall traffic. which is forecasted to continue. According to Cisco, companies can expect to see their network traffic triple by 2022. This will require organizations to make a proportional increase in data storage and maintain a brute force, record-everything approach for network forensics that will cost companies significantly more in terms of time and money. This runs counter to most companies' digital transformation journeys where the bigger objective is to save on operational costs, increase IT agility, and improve responsiveness.
Fortunately, full packet capture is finally entering the age of practicality because of the introduction of AIOps. Gartner defines AIOps as the application of machine learning (ML) and data science to IT operations problems. The firm also predicts that large enterprises use of AIOps tools will reach 30% by 2023. The adoption of AIOps will pave the way to security automation like intelligent packet capture. This is an exciting development that our company is pursuing, along with others in the industry, to enable security analysts to utilize AIOps for network forensics.
The advancement of machine learning (ML) and artificial intelligence (AI) is enabling new innovations in full packet capture to bring some needed relief to the security analyst. When a machine learning engine 'learns' to classify packets to predict those that need to be recorded, the security analyst benefits by having data with higher fidelity allowing he or she to conduct more meaningful and expedient forensics. As noted security analyst and trainer, Chris Sanders says in his blog post, "if you can distill a PCAP down to key events then you'll have a much more manageable set of data points to aid your investigation."
Thanks to AIOps, security analysts now have an opportunity to utilize more open source technologies and experiment with ML and AI to make packet capture work better for them and their organizations. Before it was unrealistic to expect a group of analysts in a security operations center to proactively ferret through petabytes of data in search of an anomaly or indicator of compromise in a timely manner. Normally, this would be — at best — a week-long exercise without ML or AI. Access to these enabling technologies represent a significant improvement in the state of full packet capture, making them practical and invaluable resources for security analysts.
Related Content:
Check out The Edge, Dark Reading's new section for features, threat data, and in-depth perspectives. Today's top story: "5 Disruptive Trends Transforming Cybersecurity."
About the Author
You May Also Like
The State of Attack Surface Management (ASM), Featuring Forrester
Nov 15, 2024Applying the Principle of Least Privilege to the Cloud
Nov 18, 2024The Right Way to Use Artificial Intelligence and Machine Learning in Incident Response
Nov 20, 2024Safeguarding GitHub Data to Fuel Web Innovation
Nov 21, 2024