Multiparty Encryption Allows Companies to Solve Security-Data ConundrumMultiparty Encryption Allows Companies to Solve Security-Data Conundrum
An interdisciplinary research team constructs a way for companies to share breach data without revealing specific details that could exposes businesses to legal risk.
September 9, 2020
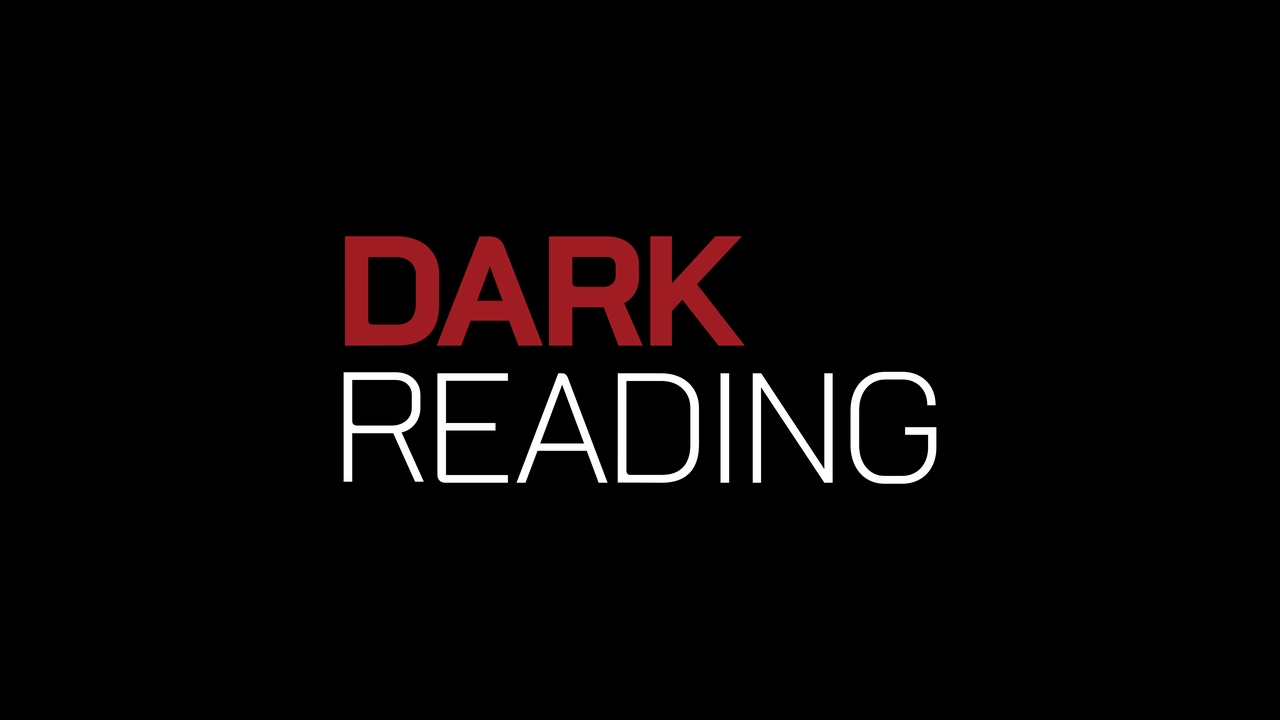
A system that allows companies to submit breach data anonymously and then benefit from the aggregate statistics for their industries could give executives and policymakers a more accurate understanding of how breaches impact businesses and give companies the timely threat intelligence they need to prepare for attacks.
The Secure Cyber Risk Aggregation and Measurement (SCRAM) system — created by an interdisciplinary team of policy, financial, and computer-science researchers at the Massachussetts Institute of Technology (MIT) — uses a special type of encryption to allow various calculations to be performed on protected data in the context of a multiparty computation (MPC) system. An initial proof-of-concept trial not only delivered aggregate breach data for a group of six companies, but it also collected information about the adoption rate of security controls and the controls blamed for the greatest loss.
The researchers plan to next conduct a larger trial of the technology with 60 to 70 companies in several industries to gather sector-specific data, says Taylor Reynolds, technology policy director of MIT's Internet Policy Research Initiative.
"We have shown that firms are willing to share this really sensitive data as long as they know it is going to be protected," he says. "And what that does is it opens up a whole new set of data and statistics for us that will allow us better to better defend our networks."
The research could solve one of the most enduring problems of cybersecurity: the lack of good data on breaches and information on what controls are working. While several industries — most notably healthcare — are required to disclose information on cybersecurity incidents, the practice remains relatively uncommon and minor cybersecurity events have always been underreported.
A privacy-preserving system could solve the major hurdle preventing such sharing of data, says Darren Van Booven, lead principal consultant at security-services firm Trustwave.
"One of the things that I've always noticed over the course of my career is the difficulty in being able to get quality information on what works and what doesn't, what have other organizations found to be more effective in the way of controls, and what exactly are the losses that have been occurred," he says. "This impacts the job of every CISO because they are trying to report to their executive leadership on what exactly the real risk to their company is right now."
The idea for the system came out of interviews with executives in critical-infrastructure industries, such as financial, oil and gas, and the electric industries. Each industry wanted data, but no executive wanted to put their business at risk by acknowledging breaches, says MIT's Reynolds
"One of the messages that kept coming out was they needed a better way to share data and share information because the current methods are not working," he says. "We put our minds together and knew we had the pieces ... let's get together and devise a way that firms can share data securely without having to reveal it or disclose it to anyone else."
The group of researchers created an MPC system that preserves privacy. The system is enabled by a special type of encryption that allows some types of math to be performed on the encrypted values. Known as threshold homomorphic encryption, the technique is a special way of protecting data by allowing each party to encrypt the information and then decrypt the results of any aggregate calculation.
The technique solves two problems with other methods of aggregation. Take, for example, a gathering of people who wants to share information on salaries. They could give all the information to a trusted third party, which could then do the calculations and provide an average income for the group. The third party, however, could be compromised or, in the end, found untrustworthy, resulting in a leak of information on a specific person's salary — a violation of privacy. Alternatively, the group could put all the information into a hat and then aggregate the data, but participants could potentially be identified from just knowing the details of any single incident.
However, if each participant added a large random number to their salary, then passed along the total to the next person, no individual salary would be compromised. In a second round of calculation, each person could subtract the large random amount they had previously added, resulting in the exact sum of their incomes.
"Nowhere along that path did anyone have to reveal their own salary in order for us to run that computation," Reynolds says. "It is that type of mathematical modeling that allows us to run those computations on the platform."
The SCRAM system uses a similar approach with homomorphic encryption, a type of privacy-preserving cryptography that allows calculations on encrypted data.
The pilot project collected data on more than 49 security incidents from the six large private-sector firms and the specific security-control failures that the companies blamed for each incident. Centralized log management was the top control failure linked to breaches, associated with almost $6 million in aggregate losses over the 49 security incidents.
Future trials will attempt to structure the questions and answers to reveal stronger links between controls and breach damages, says Reynolds.
"The Holy Grail here is trying to understand return on investment of security controls," he says. "If I spend the money on X, what will be the return on investment that I get on that when I do risk modeling?"
With the privacy-preserving system, such data may no longer be out of reach.
About the Author
You May Also Like