New Research Seeks to Shorten Attack Dwell Time
It can take months for an organization to know they've been hacked. A new DARPA-funded project seeks to reduce that time to hours.
May 18, 2018
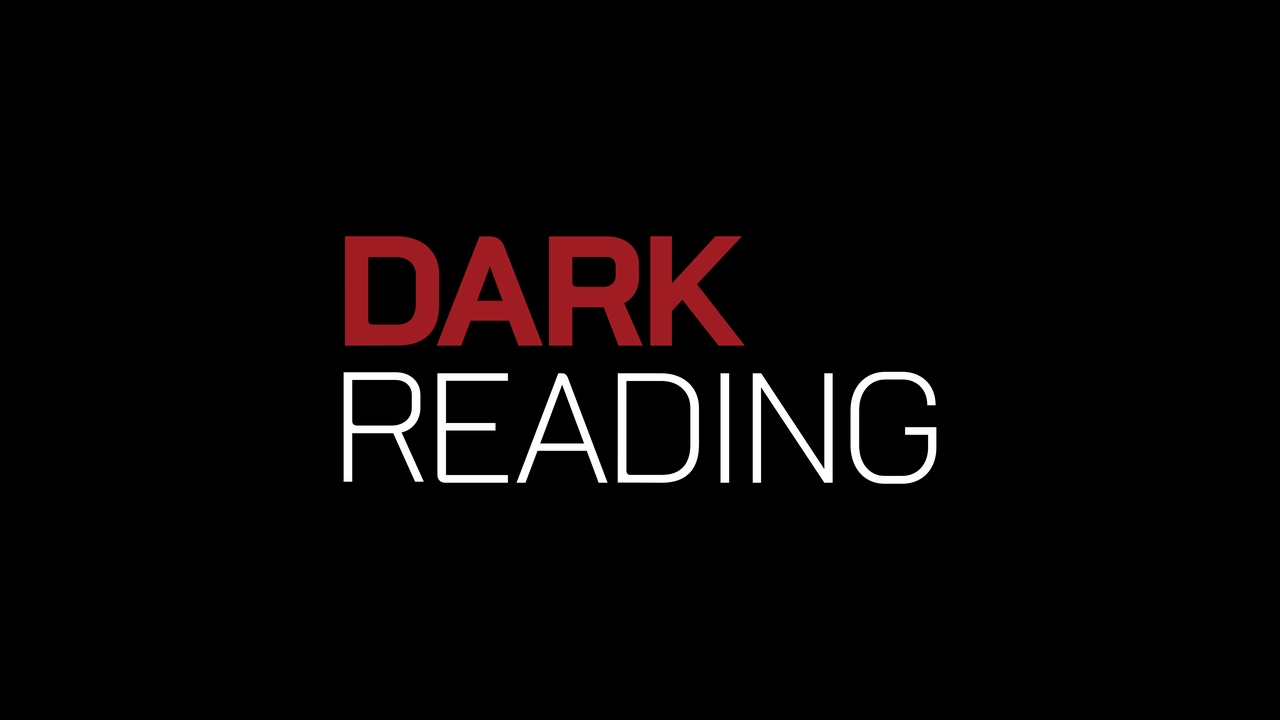
One of the major issues in IT security is not that attacks and exploits are successful. It's that they're successful and then go unnoticed for so long. A new DARPA-funded research project at Georgia Tech is applying a variety of techniques to dramatically reduce the "dwell time" of an attack from the current average of more than 6 months to as little as 24 hours.
The DARPA grant gives a team of researchers $12.8 million over four years for the "Gnomon" project. Gnomon's approach is based on the admission that breaches are inevitable. What the project aims to do is examine the behavior of the devices and systems attached to the network to tell when something begins to exhibit suspicious behavior and allow professionals or automated systems to immediately begin remediation.
Gnomon's operation doesn't rely on identifying malicious files at all. "We're not looking at the malware, because they might not use malware, they might use something like Powershell," says co-principle investigator Manos Antonakakis, an assistant professor in Georgia Tech’s School of Electrical and Computer Engineering. Instead, the behavior-based tactics seek to mitigate one of the attackers' primary advantages over defenders.
"One study we did last year showed that threats are live in the wild for many months before we even get a sample," says Antonakakis. The study says, "…the PUP [potentially undesirable program]-related domains are active an average of 192 days before we get to dynamically analyze the corresponding samples."
The sheer number of domains (and therefore malware families) profiting from a delay in analysis is huge. Again, from the research for the study, "…302,953 malware domains were active at least two weeks — in some cases many months — before the corresponding malware samples were analyzed."
Analyzing behavior of large networks in real time requires massive compute power. In Gnomon's case, that power is used in service to "dynamic intelligence." Asked to define dynamic intelligence and differentiate it from machine intelligence or AI, Antonakakis says, "Dynamic intelligence is based on the dynamic modeling concept. You can build models that can characterize both short term and long term behavior." The dynamic model that looks at object behavior over time is critical to the analysis.
Once malicious behavior has been identified, network security professionals must still figure out what to do about it, for example, whether blackhole, honeypot, system rebuild, file remediation, or some other action is warranted. Antonakakis says that his researchers gained insight into the answers when doing work on how best to take down botnets. The goal for Gnomon is straightforward if initially counter-intuitive; make malware more complicated.
"What this project will do in 3 - 4 years is that it increases the load for the adversaries; it makes them work harder," Antonakakis says. "If they do that, they have to engage in more complicated attacks, and that increases the chance that they will make mistakes that will help us identify them earlier."
In addition, complicated malware and malicious activity tends to stand out more vividly than does simple software. Today, Gnomon is working with two unnamed U.S. telecommunications companies to analyze networks and pass along information. "You need to study the domain knowledge you have about the threats multiple times through the day, and do that across the networks you're protecting," Antonakakis says. "Our goal is to be able to detect a change in behavior in under 24 hours."
Related Content:
About the Author
You May Also Like