Using Adversarial Machine Learning, Researchers Look to Foil Facial Recognition
For privacy-seeking users, good news: Computer scientists are finding more ways to thwart facial and image recognition. But there's also bad news: Gains will likely be short-lived.
July 9, 2020
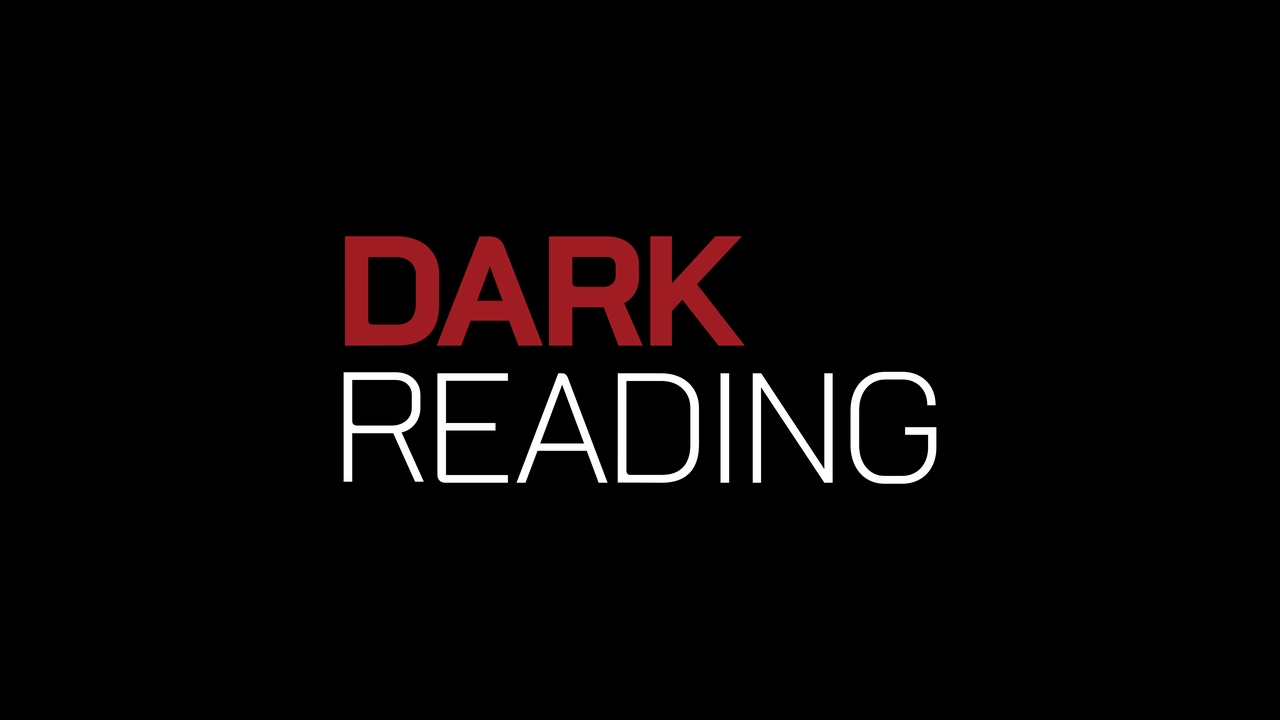
Suspect identification using massive databases of facial images. Reputational attacks through deep fakes videos. Security access using the face as a biometric. Facial recognition is quickly becoming a disruptive technology with few limits imposed by privacy policy.
Academic researchers, however, have found ways to — at least temporarily — cause problems for certain classes of facial-recognition algorithms, taking advantages of weaknesses in the training algorithm or the resultant recognition model. Last week, a team of computer-science researchers at the National University of Singapore (NUS) published a technique that locates the areas of an image where changes can best disrupt image-recognition algorithms, but where those changes are least noticeable to humans.
The technique is general in that it can be used to develop an attack against other machine-learning (ML) algorithms, but the researchers only developed a specific instance, says Mohan Kankanhalli, a professor in the NUS Department of Computer Science and co-author of a paper on the adversarial attack.
"Currently, we need to know the class [of algorithm] and can develop a solution for that," he says. "We are working on its generalization, to have one solution that works for every class, current and future. However, that is nontrivial and hence we anticipate it will take time."
The research raises the possibility of creating photos that people can easily perceive but that foils commonly used facial-recognition algorithms. Turned into a filter, for example, the technique could allow users to add imperceptible changes to photos to make them more difficult for ML algorithms to classify and foil the development of reverse image search engines.
Such methods, however, currently take advantage of the brittleness of training algorithms. As ML-focused companies develop more robust algorithms, privacy-seeking Internet users will have to decide whether to degrade photos to the point where the changes are noticeable to humans.
"It's a very difficult problem because pictures themselves have utility for us," says Joey Bose, a PhD student in computer science at McGill University, who published related adversarial ML research in 2018. "But the only surefire way to guarantee privacy is to remove content from the image, and if you remove content, it becomes less useful."
The developments come as several companies are using — some would say abusing — the plethora of image content readily available on the Internet. Social media sites make it easy to gather an enormous number of images on which to train neural networks, allowing companies to create sophisticated recognition models or turn people's photos into deep-fake videos. Clearview.ai, a company that sells its ability to find people's online presence from a provided photo, collected hundreds of millions of photos from social media sites to create its large reverse image search engine.
The NUS technique has to be tailored for the specific class of facial recognition, which means the researchers have to know the details of the facial-recognition system. Only then can they create a technique for confounding that approach.
While such techniques could help people protect their images and their privacy, most of the research is aimed at preventing such attacks in the future: Far from just attacking ML algorithms, the researchers are basically performing the role of a red team, checking the quality of the models, says McGill's Bose.
"The research can inform policy and help companies know what they need to check off before these systems are put into the wild," he says. "Better models are more reliable."
And even if the researchers did find an approach that could foil the most common approaches to facial recognition, the surveillance system makers would likely be able to find ways to work around the technology, says the National University of Singapore's Kankanhalli.
Any success will be "temporary in the sense it is like a cat-and-mouse game," Kankanhalli explains. "There will be better techniques developed by the data harvesters, and in response there will be better privacy techniques to counter them. There probably will never be a final solution in such constantly evolving problem areas."
Unlike encryption, which can provide privacy even against well-funded adversaries, ML attacks often only work until the model is retrained, he says. The best way to limit artificial intelligence is through policy, Kankanhalli says.
"When consumers frown upon companies who violate privacy, then there will be a backlash against them, which will change the behavior of such companies," he says. "We as consumers and users should therefore articulate what is acceptable and what is not. I strongly believe we need work on all these three fronts to tackle this data harvesting problem."
Related Content:
About the Author
You May Also Like
Applying the Principle of Least Privilege to the Cloud
Nov 18, 2024The Right Way to Use Artificial Intelligence and Machine Learning in Incident Response
Nov 20, 2024Safeguarding GitHub Data to Fuel Web Innovation
Nov 21, 2024The Unreasonable Effectiveness of Inside Out Attack Surface Management
Dec 4, 2024