Fighting AI-Powered Fraud: Let the Battle of the Machines Begin
As cybercriminals tap the power of machine learning and generative AI to outwit fraud-detection systems, online fraud-prevention technologies must evolve accordingly.
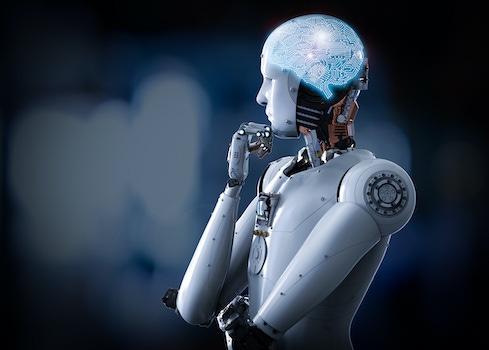
In 2022, US banks processed more than $448 billion in peer-to-peer (P2P) transactions, making these platforms a prime target for scammers. As is the case with "classic" fraud paradigms such as phishing, Zelle or Venmo fraud is increasingly being fueled by artificial intelligence (AI). As cybercriminals tap the power of machine learning (and soon enough) generative AI to outwit fraud-detection systems, online fraud-prevention technologies must evolve accordingly.
Introducing AI Into the Online Fraud Landscape
The rapid pace of digital transformation has benefited our society and economy immeasurably. It's also been a huge boon to cybercriminals, who, like the companies they target, use cloud infrastructure every day to scale their operations. Leveraging the power of the cloud, new malicious machine learning (ML) models offer the prospect of automating tasks that only humans could perform a few years ago.
As a result, the next wave of innovation in fraud features cloud-powered AI — or more correctly, ML, effectively making modern fraud prevention a "battle of the machines." These battles usually start with fraudsters making the first move, using cloud services to build ML models capable of circumventing the defenses built by companies to spot obvious fraud.
How AI Can Help Fool Fraud-Detection Systems
Consider a typical fraud-mitigation system in a retail setting. Say a company sets a rule that in certain locations, transactions over $900 are automatically flagged for secondary verification. An ML tool could be programmed to calculate through trial and error the point at which high-value transactions are inspected. Then the adversary need only ensure their fraudulent transactions stay under $900 and are based in the right geolocation to avoid detection. What was once a time-consuming process becomes a simple matter of cloud-powered analytics.
Even sophisticated ML models can be probed and attacked for weaknesses by malicious AI. The more opaque AI systems become, the riskier they are to deploy in production settings. Humans will only have a limited understanding of their behavior and the outputs they might generate. Plus, to remain effective, they need to be trained on data from previous attacks. This combination make them vulnerable to exploitation when presented with a slightly different scenario. It only takes some targeted trial and improvement for malicious AI to learn those oversights and blind spots.
That's not all. AI could also generate fake image data of a user's face that's compelling enough to allow a transaction to proceed, as the checking computer assumes it to be a photo of a new user. Or it could be trained with public video or audio data (for example, clips posted to social media) to impersonate legitimate customers in authentication checks. Similarly, AI could be trained to mimic human behavior such as mouse movements to outwit machines designed to spot signs of non-human activity in transactions. It could even generate different combinations of stolen data to bypass validation checks — a compute-intensive task that can be solved using the public cloud.
How Defenders Can Strike Back
Cybercriminals often have an advantage over defenders, and that is currently the case for online fraudsters leveraging AI. They have the element of surprise and the financial motivation to succeed. Yet fraud and risk teams can counter malicious AI by tweaking their own approaches. AI can be trained by the bad guys to mimic human behavior more realistically. But if it's used in automated attacks, it will still need to be deployed like a bot, which can be detected by tweaking and innovating fraud-detection algorithms.
Not only can defenders bolster their defense by deploying new and improved ML algorithms, they can also change the battlefield to one that provides them with a strategic advantage. For example, by shifting fraud detection to the network edge, much closer to the devices used to make online transactions, defenders create a dynamic where unusual or high-risk behavior is easier to spot with a higher degree of accuracy.
Using existing infrastructure, such as content delivery networks (CDNs), fraud detection can move to the edge in a relatively seamless manner. Moving fraud detection to the edge not only provides a much clearer and more detailed view of a user's online experience (or "customer journey," as industry wonks like to call it), it also creates a richer and nuanced baseline, making it easier to spot and thwart malicious AI.
By capturing intelligence across the user's entire session, there's more opportunity to spot machine-generated anomalies. Flexible signal generation can also be a powerful tool in a security engineer's arsenal. It could be used in the examples above to trigger image analysis as soon as an image is uploaded. Or to compare mouse movements across non-financial transaction pages with those where a financial transaction is being initiated. Furthermore, from a strategic standpoint, greater visibility into the customer experience is sure to provide valuable insights that can support other business functions.
AI is becoming very sophisticated very quickly. Moving fraud detection to the edge is a preemptive move that can make it harder for fraudsters to succeed, increasing the likelihood that they'll simply move on to more vulnerable targets. Either way, the skillful application of cloud-based ML models to both commit and defend against online fraud heralds the beginning of a new, cloud-native, AI-driven arms race.
Buckle up, the battle of the machines has begun.
About the Author
You May Also Like