Responsibly Implementing AI, the Unstoppable Force
Balancing the good and bad of AI/ML means being able to control what data you're feeding into AI systems and solving the privacy issues to securely enable generative AI.
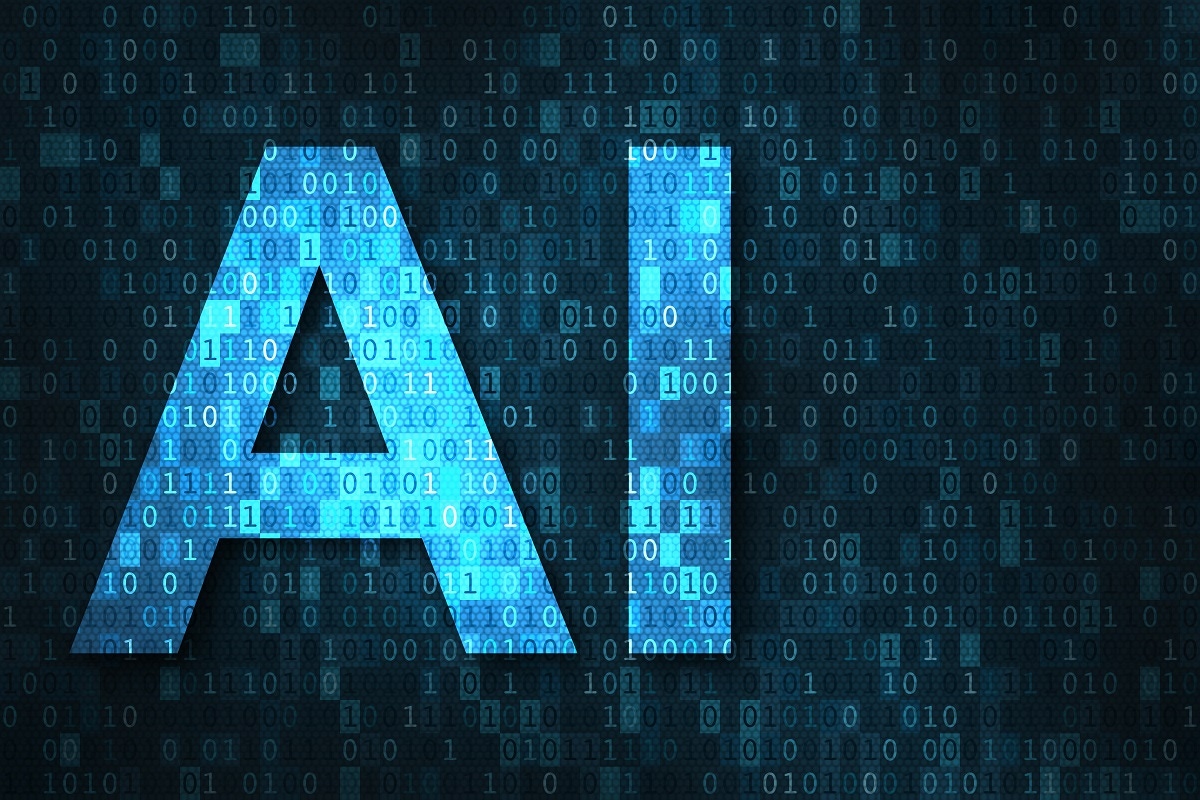
COMMENTARY
Lately, at least half of C-suite leaders I meet with want to talk about artificial intelligence and machine learning (AI/ML), how their companies can enable it, and whether safe enablement is even possible. One leader at a large financial firm recently told me the board is very eager to leverage generative AI: "It's a competitive advantage. It's the key to automation. We have to start using it." But when I asked what they're doing with AI, they replied, "Oh, we're blocking it."
Years ago, there was buzz about the cloud's immediate benefits and transformative use cases but also pervasive resistance to adoption because of potential risks. Ultimately it was impossible to try to stop end users from using cloud-based tools. Everybody eventually said, "OK, we've got to find ways to use them," because the benefits and flexibility far outweighed the security risks.
History is now repeating itself with AI, but how do we securely enable it and control sensitive data from exposure?
The Good News About AI
People (more than organizations) are using generative AI to see information in a more conversational way. Generative AI tools can listen and respond to voice input, a popular alternative to typing text into a search engine. In some forward-thinking organizations, it's even being applied to automate and innovate everyday tasks, like internal help desks.
It's important to remember that many of the most important and exciting use cases are not actually coming from generative AI. Advanced AI/ML models are helping solve some of the biggest problems facing humanity — things like developing new drugs and vaccines.
Enabling customers in the healthcare, medical, and life sciences fields to securely implement AI means helping them solve those big problems. We have nearly 100 data scientists working on AI/ML algorithms every day, and we have released more than 50 models in support of stopping threats and preventing exfiltration of sensitive data from insiders or attackers who have infected insiders.
Security problems that were intractable are now solvable using AI/ML. For example, attackers have been stealing sensitive data in innovative ways, lifting secrets from virtual whiteboards or concealing data in images by emailing images embedded with sensitive information to evade common security tools. An attacker could access an exposed repository with credit card images that are hazy or have a glare that traditional security may not recognize, but advanced ML capabilities could help catch. These kinds of sophisticated attacks, enabled using AI/ML, also cannot be stopped without the use of AI/ML.
The Bad News About AI
Every technology can be used for good or for bad. Cloud today is both the biggest enabler of productivity and the most frequently employed delivery mechanism for malware. AI is no different. Hackers are already using generative AI to enhance their attack capabilities — developing phishing emails or writing and automating malware campaigns. Attackers don't have much to lose nor to worry about how precise or accurate the results are.
If attackers have AI/ML in their arsenal and you don't, good luck. You must level the playing field. You need tools, processes, and architectures to protect yourself. Balancing the good and bad of AI/ML means being able to control what data you're feeding into AI systems and solving the privacy issues to securely enable generative AI.
We are at an important crossroads. The AI Executive Order is welcome and necessary. While its intention is to give guidance to federal agencies on AI systems testing and usage, the order will have ample applicability to private industry.
As an industry, we must not be afraid to implement AI and must do everything possible to thwart bad actors from applying AI to harm industry or national security. The focus must be on crafting a framework and best practices for responsible AI implementation, specifically when it comes to generative AI.
Plot a Path Forward
Here are four key points of consideration to help plot a path forward:
Realize that generative AI (and AI/ML in general) is an unstoppable force. Don't try to stop the inevitable. Accept that these tools will be used at your organization. It's better if business leaders shape the policies and procedures of how it happens, rather than attempt to outright block their use.
Focus on how to use it responsibly. Can you ensure your users are accessing solely corporate versions of generative AI applications? Can you control whether sensitive data is shared with these systems? If you can't, what steps can you take to improve your visibility and control? Certain modern data security technologies can answer these questions and help provide a framework to manage it.
Don't forget about efficacy. This means the precision and accuracy of its output. Are you sure the results from generative AI are reliable? AI doesn't remove the need for data analysts and data scientists — they will be invaluable in helping organizations assess efficacy and accuracy in the coming years as we all reskill.
Classify how you use it. Some applications will require high precision and accuracy as well as access to sensitive data, but others will not. Generative AI hallucinations in a medical research context would deter its usage. But error rates in more benign applications (like shopping) may be OK. Classifying how you're using AI can help you target the low-hanging fruit — the applications that aren't as sensitive to the tools' limitations.
It's also fair to say that there's a lot of AI-washing out there. Everybody's proclaiming, "We're an AI company!" But when the rubber hits the road, they have to use it, they have to implement it, and it has to provide value. To responsibly achieve any of those aspirational outcomes from generative AI or broader AI/ML models, organizations must first ensure they can protect their people and data from the risks inherent to these powerful tools.
About the Author
You May Also Like