Using AI to Break Detection Models
Pitting machine learning bots against one another is the new spy vs. spy battle in cybersecurity today.
July 25, 2017
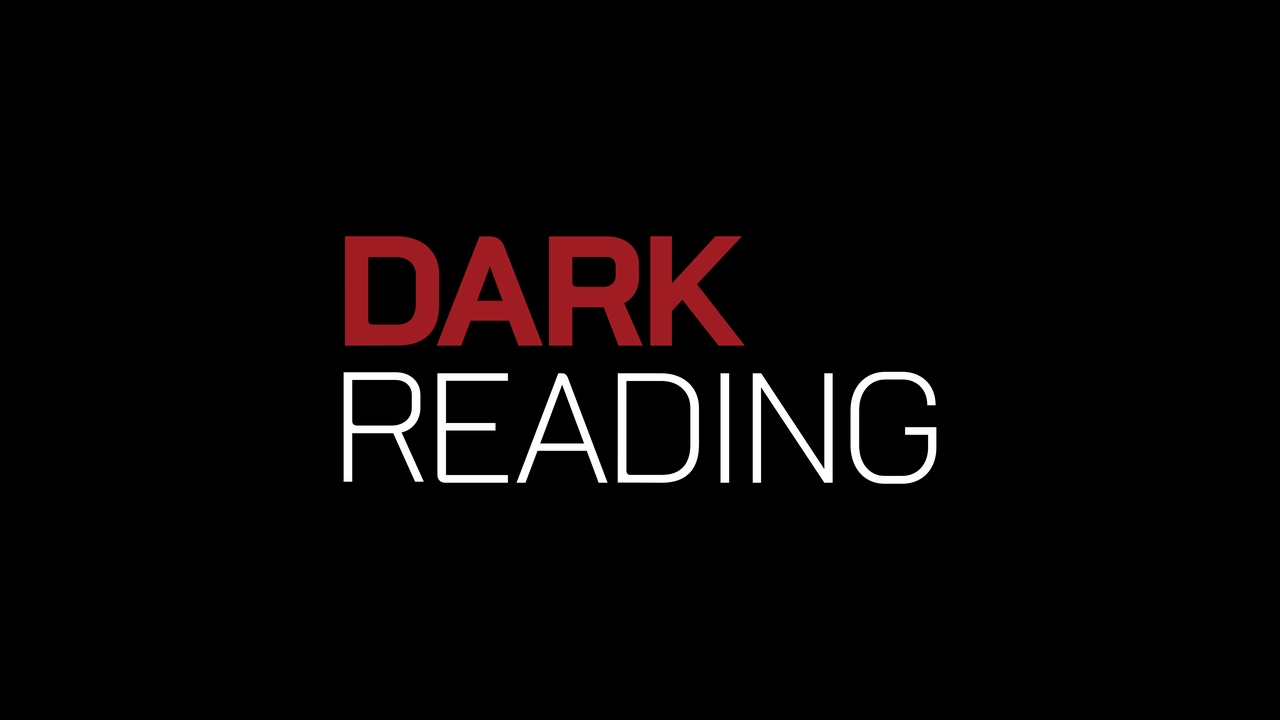
In the spy versus spy world that pits cybersecurity defense against money-motivated attackers, it always pays to think a few steps ahead of the attackers. Any security pro who has seen the direction that detection is going these days with artificial intelligence and machine learning understands that those next attacks steps are probably going to involve some sort of subversion of the AI algorithms. If security wants to effectively use AI, it will need to find ways to harden those models
This week at Black Hat, one researcher hopes to contribute to the discipline by showing off a new automated AI agent that probes the data science behind machine learning malware detection models and looks for mathematical weaknesses.
"All machine learning models have blind spots. All of them. And a sophisticated and motivated adversary is out there trying to exploit them," says Hyrum Anderson, technical director of data science for Endgame. "We have created an artificial agent that tries to automatically discover those blind spots."
As he puts it, the agent "literally plays a game against our model and tries to beat it," essentially automating the auditing of the mathematical underpinning of detection mechanisms. The agent essentially inspects an executable file and uses a sequence of file mutations to test the detection model. This agent uses its own brand of machine learning to figure out which sequences of mutations are most likely to create a variant that evades the model. Using the information it gains from this automated test, the agent can create a policy for developing malware variants that have a high likelihood of breaking the opposing machine learning model of the detection engine.
This is the logical next step in advancement of thought about cybersecurity's auditing of machine learning efficacy, and one which Anderson hopes to encourage across the industry as vendors further refine their machine learning mechanisms.
"You'll get no criticisms from me (about the competition). I think in general my colleagues and competitors are all paranoid and are always thinking about how to make (their models) secure. But that's usually a manual process. It's spot-checking and it's somebody looking at it," he says. "We wanted to take that to the next level. I don't believe that our adversaries are yet using this level of sophistication we are proposing in our research, but that's the point. We want to get there before they do."
At the moment, Anderson's use of the agent begins and ends with Endgame's own machine learning model. But, in concert with his presentation, he and his team are going to release code that is generic and adaptable for other vendors and researchers to inspect their own models.
"We want to put it out there because a rising tide raises all boats," Anderson says.
The idea of machine learning and AI hardening is generally gaining momentum with data scientists and security specialists of late. In fact, this is one of several talks at Black Hat this year that will focus on problems that can arise through flawed machine learning algorithms. For example, in one talk a data scientist with Sophos will discuss how bad data can screw up detection models. Another from a group of Georgia Tech researchers will dive into a new tool that aims to sabotage detection mechanisms in Android antivirus apps.
Related Content:
Read more about:
Black Hat NewsAbout the Author
You May Also Like