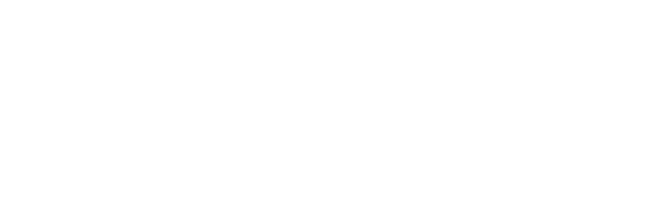
News, news analysis, and commentary on the latest trends in cybersecurity technology.
Will the AI Arms Race Lead to the Pollution of the Internet?Will the AI Arms Race Lead to the Pollution of the Internet?
Content creators want to protect their intellectual property from AI by poisoning data. Could this destroy the machine learning ecosystem?
September 1, 2023
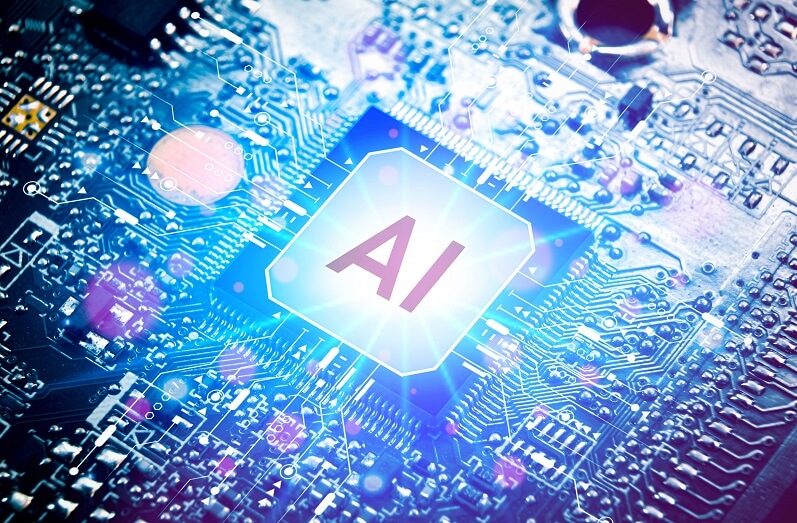
The arms race between companies focused on creating artificial intelligence (AI) models by scraping published content and creators who want to defend their intellectual property by polluting that data could lead to the collapse of the current machine learning ecosystem, experts warn.
In an academic paper published in August, computer scientists from the University of Chicago offered techniques to defend against wholesale efforts to scrape content — specifically artwork — and to foil the use of that data to train AI models. The outcome of the effort would pollute AI models trained on the data and prevent them from creating stylistically similar artwork.
A second paper, however, highlights that such intentional pollution will coincide with the overwhelming adoption of AI in businesses and by consumers, a trend that will shift the makeup of online content from human-generated to machine-generated. As more models train on data created by other machines, the recursive loop could lead to "model collapse," where the AI systems become dissociated from reality.
The degeneration of data is already happening and could cause problems for future AI applications, especially large language models (LLMs), says Gary McGraw, co-founder of the Berryville Institute of Machine Learning (BIML).
"If we want to have better LLMs, we need to make the foundational models eat only good stuff," he says. "If you think that the mistakes that they make are bad now, just wait until you see what happens when they eat their own mistakes and make even clearer mistakes."
The concerns come as researchers continue to study the issue of data poisoning, which, depending on the context, can be a defense against unauthorized use of content, an attack on AI models, or the natural progression following the unregulated use of AI systems. The Open Worldwide Application Security Project (OWASP), for example, released its Top 10 list of security issues for Large Language Model Applications on Aug. 1, ranking the poisoning of training data as the third most significant threat to LLMs.
A paper on defenses to prevent efforts to mimic artist styles without permission highlights the dual nature of data poisoning. A group of researchers from the University of Chicago created "style cloaks," an adversarial AI technique of modifying artwork in such a way that AI models trained on the data produce unexpected outputs. Their approach, dubbed Glaze, has been turned into a free application in Windows and Mac and has been downloaded more than 740,000 times, according to the research, which won the 2023 Internet Defense Prize at the USENIX Security Symposium.
While he hopes that the AI companies and creator communities will reach a balanced equilibrium, current efforts will likely lead to more problems than solutions, says Steve Wilson, chief product officer at software security firm Contrast Security and a lead of the OWASP Top-10 for LLM Applications project.
"Just as a malicious actor could introduce misleading or harmful data to compromise an AI model, the widespread use of 'perturbations' or 'style cloaks' could have unintended consequences," he says. "These could range from degrading the performance of beneficial AI services to creating legal and ethical quandaries."
The Good, the Bad, and the Poisonous
The trends underscore the stakes for firms focused on creating the next generation of AI models if human content creators are not brought onboard. AI models rely on content created by humans, and the widespread use of content without permissions has created a dissociative break: Content creators are seeking ways of defending their data against unintended uses, while the companies behind AI systems aim to consume that content for training.
The defensive efforts, along with the shift in Internet content from human-created to machine-created, could have a lasting impact. Model collapse is defined as "a degenerative process affecting generations of learned generative models, where generated data end up polluting the training set of the next generation of models," according to a paper published by a group of researchers from universities in Canada and the United Kingdom.
Model collapse "has to be taken seriously if we are to sustain the benefits of training from large-scale data scraped from the web," the researchers stated. "Indeed, the value of data collected about genuine human interactions with systems will be increasingly valuable in the presence of content generated by LLMs in data crawled from the Internet."
Solutions Could Emerge ... or Not
Current large AI models — assuming they win legal battles brought by creators — will likely find ways around the defenses being implemented, Contrast Security's Wilson says. As AI and machine learning techniques evolve, they will find ways to detect some forms of data poisoning, rendering that defensive approach less effective, he says.
In addition, more collaborative solutions, such as Adobe's Firefly — which tags content with digital "nutrition labels" that provide information about the source and tools used to create an image — could be enough to defend intellectual property without overly polluting the ecosystem.
These approaches, however, are "a creative short-term solution [but are] unlikely to be a silver bullet in the long-term defense against AI-generated mimicry or theft," Wilson says. "The focus should perhaps be on developing more robust and ethical AI systems, coupled with strong legal frameworks to protect intellectual property."
BIML's McGraw argues that the large companies working on LLMs today should invest heavily in preventing the pollution of data on the Internet and that it is in their best interest to work with human creators.
"They are going to need to figure out a way to mark content as 'we made that, so don't use it for training.' Essentially, they may just solve the problem by themselves," he says. "They should want to do that. ... It's not clear to me that they have assimilated that message yet."
About the Author
You May Also Like
Shifting Left: DevSecOps in the Cloud
Feb 4, 2025Uncovering Threats to Your Mainframe & How to Keep Host Access Secure
Feb 13, 2025Securing the Remote Workforce
Feb 20, 2025Emerging Technologies and Their Impact on CISO Strategies
Feb 25, 2025How CISOs Navigate the Regulatory and Compliance Maze
Feb 26, 2025