Expect an Increase in Attacks on AI SystemsExpect an Increase in Attacks on AI Systems
Companies are quickly adopting machine learning but not focusing on how to verify systems and produce trustworthy results, new report shows.
April 27, 2021
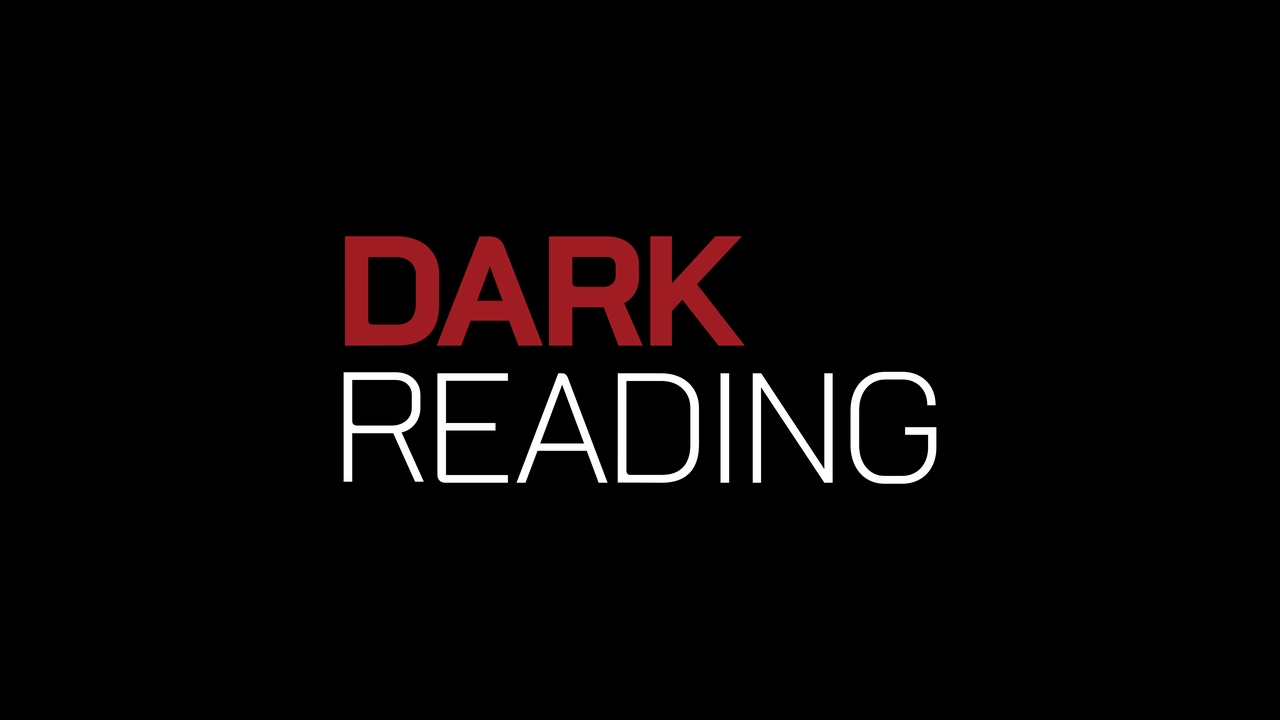
Research into methods of attacking machine-learning and artificial-intelligence systems has surged—with nearly 2,000 papers published on the topic in one repository over the last decade—but organizations have not adopted commensurate strategies to ensure that the decisions made by AI systems are trustworthy
A new report from AI research firm Adversa looked at a number of measurements of the adoption of AI systems, from the number and types of research papers on the topic, to government initiatives that aim to provide policy frameworks for the technology. They found that AI is being rapidly adopted but often without the necessary defenses needed to protect AI systems from targeted attacks. So-called adversarial AI attacks include bypassing AI systems, manipulating results, and exfiltrating the data that the model is based on.
These sorts of attacks are not yet numerous, but have happened, and will happen with greater frequency, says Eugene Neelou, co-founder and chief technology officer of Adversa.
"Although our research corpus is mostly collected from academia, they have attack cases against AI systems such as smart devices, online services, or tech giant's APIs," he says. "It's only a question of time when we see an explosion of new attacks against real-world AI systems and they will become as common as spam or ransomware."
Research into adversarial attacks on machine learning and AI systems has exploded in recent years, with more than 1,500 papers on AI security published in 2019 on the scientific publishing site ArXiv.org, up from 56 in 2016, according to Adversa's Secure and Trusted AI report.
Yet, that is only a single type of threat. Adversarial attacks on AI systems may be the largest case—and it's certainly the one garnering the most attention—but there are other major cases as well, says Gary McGraw, co-founder and director of the Berryville Institute of Machine Learning (BIML). The group of machine-learning researchers at BIML identified 78 different threats to machine-learning models and AI systems. Top threats also include data poisoning, online system manipulation, attacks on common ML models, and data exfiltration, according to the BIML report, An Architectural Risk Analysis of Machine Learning Systems.
Late last year, Mitre, Microsoft, and other organizations—including BIML—released the Adversarial ML Threat Matrix, which includes 16 categories of threats.
"One of the things you should do right off the bat is to familiarize yourself with those risks, and think about whether any of those risks affect your company," McGraw says. "If you don't think about them while you are coding up you ML systems, you are going to be playing catch up later."
Image Problems
The variety of potential attacks is staggering. To date, however, researchers have focused mainly on attacking image-recognition algorithms and other vision-related machine learning models, with 65% of adversarial machine-learning papers having a vision focus, according to the Adversa analysis. In July, for example, researchers found a variety of ways to attack facial recognition algorithms. The other third of papers focused on analytical attacks (18%), language attacks (13%), and the autonomy of the algorithms (4%), according to Adversa.
The popularity of using adversarial machine learning to undermine image- and video-related algorithms is not because other applications of machine learning are less vulnerable, but because the attacks are, by definition, easier to see, Adversa stated in the report.
"Image data is the most popular target because it is easier to attack and more convincing to demonstrate vulnerabilities in AI systems with visible evidence," the report stated. "This is also correlated to the attractiveness of attacking computer vision systems due to their rising adoption."
In addition, the report also showed researchers focused on dozens of applications, with the largest share—43%—comprising image classification applications, with facial recognition and data analytics application coming in a distant second and third place with a 7% and 6% share, respectively.
Companies should raise awareness of the security and trust considerations of machine-learning algorithms with everyone involved in developing AI systems. In addition, businesses should conduct AI security assessments based on threat models, and implement continuous security monitoring of AI systems, Adversa AI's Neelou says.
"Organizations should start an AI security program and develop practices for a secure AI lifecycle," he says. "This is relevant regardless of whether they develop their own AIs and use external AI capabilities."
In addition, firms should investigate the broader range of threats that affect their use of machine learning systems, says BIML's McGraw. By considering the full range of AI threat, companies will be more ready for, not just future attacks, but poorly created AI and machine-learning systems that could lead to poor business decisions.
"The more people that think about this, the better it will be for everyone," he says.
About the Author
You May Also Like